Establishing a carcinoembryonic antigen-associated competitive endogenous RNA network and forecasting an important regulatory axis in colon adenocarcinoma patients
Highlight box
Key findings
• The LCMT1-AS2/ribosomal protein S6 kinase A5 (RPS6KA5) axis may play an important role in tumor progression and is an important prognostic factor for predicting the clinical outcome of colon adenocarcinoma (COAD).
What is known and what is new?
• Growing evidence underscores the role of competitive endogenous RNA (ceRNA) networks in COAD. The CEACAM5-encoded protein, a COAD biomarker, also has significant influence via other pathways.
• A CEACAM5-associated lncRNA-miRNA-mRNA network was constructed, ultimately identifying the pivotal LCMT1-AS2/miR-454-3p/RPS6KA5 axis associated with overall survival. This axis may be instrumental in tumor progression, potentially acting as a prognostic biomarker and therapeutic target.
What is the implication, and what should change now?
• The innovation of this study is to CEACAM5-associated ceRNA networks and establish the key regulatory axis based on the ceRNA hypothesis, and this research method is relatively rare at present. In addition, the bioinformatics analysis methods we employed are relatively rich and complex, and the research idea and design are unique and innovative.
Introduction
According to the Global Cancer Statistics 2020, the morbidity of colorectal cancer (CRC) ranks as the third highest worldwide, while its mortality rate is the second leading cause of oncological fatalities globally (1). CEACAM5 encodes a cell surface glycoprotein, which represents the founding member of the carcinoembryonic antigen (CEA) protein family. CEA is synthesized in the cytoplasm and then secreted through the cell membrane into the extracellular space, entering the surrounding body fluids. CEA levels in normal adult colonic tissues and sera are usually low, but inflammatory responses and multiple solid tumors can lead to elevated serum CEA level (2,3). Serum CEA has been utilized as a broad-spectrum tumor marker and therapeutic target for various solid tumors, including CRC (4,5). Furthermore, CEACAM5 plays a role in promoting the development of colon cancer as a homophilic or specific adhesion molecule (6) and indirectly influences colon cancer development through unique characteristics recognized by the immune system (7). Mutated CEACAM5 can promote CRC carcinogenesis by modifying the gut microbiota (8).
Long non-coding RNAs (lncRNAs) have no or limited protein-coding capacity. Numerous lncRNAs are dysregulated in various types of cancer and are associated with cell proliferation, migration, apoptosis, angiogenesis, drug resistance, and poor prognosis (9). However, the functions of most lncRNAs remain unclear. The competitive endogenous RNA (ceRNA) hypothesis, which reveals a new pattern of gene expression regulation (10), provides a pathway for predicting the noncoding function of any uncharacterized RNA transcript (11). Certain lncRNAs can function as ceRNAs, harboring the same microRNA (miRNA) response elements as messenger RNAs (mRNAs). Consequently, they establish a competitive interaction for the same type of miRNAs. In this manner, lncRNAs indirectly modulate the expression levels of mRNAs, thereby orchestrating cellular functions (12). For instance, the pseudogene PTENP1 functions as a miRNA sponge to modulate the expression of PTEN, which plays a role in many cancer pathways (13).
Elucidating the molecular mechanisms of the development and progression of colon cancer and identifying promising biomarkers are critical to the identification of novel therapeutic targets and improvement of patient outcomes (14). Considering the critical role of CEACAM5, we endeavor to construct a CEACAM5-associated ceRNA network utilizing bioinformatics techniques, with the objective of identifying potential promising biomarkers or therapeutic targets. First, the clinical and gene-expression data of colon adenocarcinoma (COAD) in The Cancer Genome Atlas (TCGA) were used in identifying differential genes for colon cancer and normal tissue s and for CEACAM5 high- and low-expression groups. Then, a database software was used in predicting lncRNA-miRNA and miRNA-mRNA binding pairs, and lncRNA-miRNA-mRNA triple regulatory networks were constructed. Prognostic and correlation analyses were then performed on clinical pathological factors in the ceRNA regulatory networks, and the key LCMT1-AS2/ribosomal protein S6 kinase A5 (RPS6KA5) regulatory axis was determined. Finally, the biological mechanism of the LCMT1-AS2/RPS6KA5 axis and its possible clinical significance in colon cancer were explored from a multiomic perspective (Figure 1). We present this article in accordance with the TRIPOD reporting checklist (available at https://jgo.amegroups.com/article/view/10.21037/jgo-24-43/rc).
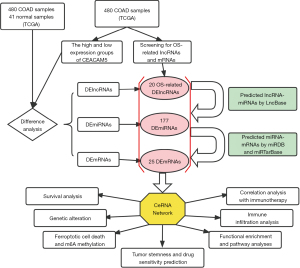
Methods
Data preparation and management
We downloaded the sequencing data of patients with COAD and clinical data from TCGA (https://portal.gdc.cancer.gov/) database. We obtained data from the University of California Santa Cruz (UCSC) Xena browser (http://xena.ucsc.edu) and compared them with healthy tissues. The somatic mutations of TCGA-COAD were downloaded and visualized using the maftools R package (15). The gene expression profiles of GSE10950 were extracted from the Gene Expression Omnibus (GEO; https://www.ncbi.nlm.nih.gov/geo/) for the verification of the analysis results. The Cancer Cell Line Encyclopedia (CCLE; https://portals.broadinstitute.org/ccle) and the Human Protein Atlas (HPA; http://www.proteinatlas.org/) databases were used in verifying gene expression in tumor cell lines at the protein level. The genomic features were studied using the cBioPortal database (http://www.cbioportal.org/). The study was conducted in accordance with the Declaration of Helsinki (as revised in 2013).
Identification of differential genes
Differentially expressed genes (DEGs) were identified using the DESeq2 (version 1.26.0) R package (16). Volcano plots and heatmaps were plotted using ggplot2 (version 3.3.3) and ComplexHeatmap R (version 2.2.0) packages, respectively. All survival analyses were conducted using the Cox proportional hazard model with R survival package (version 3.2.10).
Establishment of CEACAM5-associated ceRNA networks
According to the ceRNA hypothesis, lncRNAs in the cytoplasm can serve as natural sponges to adsorb miRNAs, thereby indirectly regulating mRNA abundance and affecting protein levels (12). First, we predicted the target-gene miRNAs (threshold =0.7) of lncRNAs by using LncBase Predicted v.2 (http://www.microrna.gr/LncBase/) and then predicted the intersection of the resulting miRNAs with differentially expressed miRNAs (DEmiRNAs). Second, the target gene mRNAs of miRNAs were predicted using miRWalk V3 version (17), which was required to satisfy miRDB (18,19) and miRTarBase (20). Similarly, the predictions were intersected with differentially expressed mRNAs (DEmRNAs) and overall survival (OS)-related mRNAs. Finally, the resulting miRNA-mRNA and lncRNA-miRNA pairs were integrated for ceRNA network construction, and the networks were plotted using Cytoscape software (https://cytoscape.org/).
Functional mechanism study
Taking the median expression of RPS6KA5 as the cutoff, we divided TCGA-COAD patients into high- and low-expression groups. Gene Ontology (GO) and Kyoto Encyclopedia of Genes and Genomes (KEGG) pathway enrichment analysis [threshold: P<0.05 or false discovery rate (FDR) <0.05] was performed using the R clusterProfiler package (version 3.18.0) (21). Further functional mechanism studies were performed. Ferroptosis and ferroptosis-related genes (FRGs) can exert oncogene and tumor suppressor effects on various cancer types (22), and most FRGs are abnormally expressed in multiple cancer types (23). In our study, 24 FRG expression differences were compared between COAD tissues and normal colon tissues, and between subgroups with different expression levels of RPS6KA5 in COAD patients. Furthermore, we evaluated the expression of m6A writers, erasers, and readers in the subgroup with the lowest RPS6KA5 expression levels and the subgroup with the highest expression levels. Malta et al. calculated DNA methylation-based stemness index (mDNAsi) and mRNA expression-based stemness index (mRNAsi) by using a logistic regression machine learning algorithm to reflect the tumor stemness, and the two stemness indexes exhibited excellent consistency in most tumors (24). We calculated mRNAsi and compared the tumor stemness of the two groups. The Genomics of Drug Sensitivity in Cancer database (GDSC; https://www.cancerrxgene.org/) was used for susceptibility analysis. We then calculated the half-maximal inhibitory concentration (IC50) values of 5-fluorouracil (5-FU) for each sample (all parameters were kept as default) through ridge regression by using the pRRophetic R package (25) and compared the two groups.
Immune infiltration and immune scores
The ESTIMATE R package (version 1.0.13) (26) was used in estimating the Immune, Stromal and ESTIMATE scores of the tumor microenvironment for each tumor sample, and then their correlation with gene expression levels was analyzed using Pearson correlation. Using the Tumor IMmune Estimation Resource (TIMER; http://timer.cistrome.org/) database, we analyzed the relationships between levels of immune cells infiltration and gene copy numbers in the ‘SCNA’ module between several immune cells and gene expression in the ‘Gene’ module and between immune cells infiltration level and COAD prognosis.
Statistics analysis
All survival analyses were performed using log-rank test and Cox regression analysis with R survival package (version 3.2.10). Wilcoxon rank sum test was used in comparing two groups, and Kruskal-Wallis test was performed in comparing more than two groups. All statistical analyses were conducted in R (R version 3.6.3). All hypothesis tests were bilateral, and the difference was considered to be statistically significant when P<0.05.
Results
Expression and prognostic value of CEACAM5 in colon cancer
CEACAM5 expression level was significantly increased in CRC and significantly higher in other solid tumors, such as lung adenocarcinoma, lung squamous-cell carcinoma, pancreatic cancer, and gastric cancer, than that in normal tissues (Figure 2A). Immunohistochemical images obtained from the HPA database also confirmed elevated CEACAM5 expression in colon cancer tissue (Figure 2B). To determine whether the gene was associated with prognosis, Cox regression was used in analyzing the correlation between genes and OS, disease-specific survival (DSS), and progression-free interval (PFI). The results suggested that the CEACAM5 high-expression group had better OS, DSS, and PFI (Figure 2C) than the low-expression group, contrary to the prognostic predictive effect of serum CEACAM5 on colon cancer (27,28).
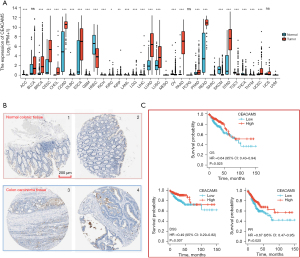
To understand the mechanisms underlying the abnormally high expression of CEACAM5 in colon cancer tissues, we analyzed the genetic mutations and copy numbers of CEACAM5 in the TCGA-COAD dataset (594 patients) on cBioPortal (Figure S1). The copy number value of CEACAM5 was positively correlated with mRNA expression (Spearman r=0.18; P<0.05). Increased gene copy number may be one of the main mechanisms causing the upregulation of CEACAM5 in CRC.
Identification of DEGs
Given the important role of CEACAM5 in colon cancer, the next steps were to construct a lncRNA-miRNA-mRNA triple regulatory network and to explore its possible clinical value. DEGs were identified in the high- and low-expression groups of CEACAM5 and between the COAD and para-tumor tissue samples from the datasets in TCGA. The threshold for lncRNA was set at |log2[fold change (FC)]| >1.5 and adjusted P value (P.adj) <0.05; miRNA threshold was set at |log2(FC)| >1 and P.adj <0.05; miRNA threshold was set at |log2(FC)| >0.7 and P.adj <0.05.
Samples with high CEACAM5 expression were compared with samples with low CEACAM5 expression. We identified 1,845 differentially expressed lncRNAs (DElncRNAs; 1 upregulated and 1,844 downregulated), 225 DEmiRNAs (0 upregulated and 225 downregulated), and 2,531 DEmRNAs (108 upregulated and 2,423 downregulated; Figure S2A-S2C). Paired differential analysis comparing COAD and matching normal colon tissues identified 2,774 DElncRNAs (2,289 upregulated and 485 downregulated), 148 DEmiRNAs (132 upregulated and 16 downregulated), and 7,561 DEmRNAs (3,980 upregulated and 3,581 downregulated; Figure S2D-S2F).
Construction of CEACAM5-associated ceRNA network
Univariate analysis of clinical and RNA-sequencing (RNAseq) data from the TCGA-COAD cohort (29) was performed using a Cox proportional risk regression model. With P<0.05 as the threshold, 464 lncRNAs and 1,479 mRNAs were identified as related to OS. Subsequently, 464 lncRNAs were intersected with the 1,845 DElncRNAs and 2,774 DElncRNAs determined from the previous analysis for production of 20 lncRNAs, which were entered into the LncBase database. MiRNAs bound to them were predicted (threshold =0.7). The resulting 212 miRNAs were intersected with 225 DEmiRNAs for the production of 177 miRNAs. Then, miRWalk was used in predicting miRNA target genes. The prediction results of miRDB and miRTarBase were used simultaneously, and 1,140 mRNAs were obtained. These mRNAs were then intersected with 7,561 DEmRNAs and 1,479 OS-associated mRNAs for the production of 25 mRNAs. Afterwards, the ceRNA networks of 18 lncRNAs, 177 miRNAs, and 25 mRNAs were visualized using Cytoscape (Figure S3).
The lncRNA LCMT1-AS2 might bind to abundant miRNAs as a ceRNA, and thus we constructed LCMT1-AS2-162miRNA-20mRNA networks (Figure 3A). Correlation analyses between LCMT1-AS2 and each mRNA revealed that LCMT1-AS2 was significantly associated with RPS6KA5, ABCB5, PRRT2, and BAHD1 (Pearson r>0.4, Figure 3B).
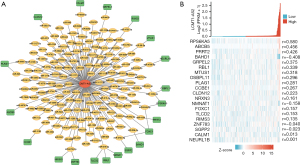
We obtained four LCMT1-AS2 regulator axes, namely, miR-4306/PRRT2, miR-4446-5p or miR-454-3p/RPS6KA5, miR-4676-5p/ABCB5 and miR-506-3p/BAHD1. Given that the cell localization of lncRNAs determined the underlying mechanisms, the subcellular localization of LCMT1-AS2 was analyzed using LNCipedia (https://lncipedia.org/) and lncLocator (http://www.csbio.sjtu.edu.cn/bioinf/lncLocator/). LCMT1-AS2 had five transcripts primarily located in the cytoplasm and cytosol (Table 1).
Table 1
Subcellular locations (score) | LCMT1-AS2:1 | LCMT1-AS2:2 | LCMT1-AS2:3 | LCMT1-AS2:4 | LCMT1-AS2:5 |
---|---|---|---|---|---|
Cytoplasm | 0.234449659 | 0.265325317 | 0.762889736 | 0.492690767 | 0.234449659 |
Nucleus | 0.087777402 | 0.098903241 | 0.176458993 | 0.383300021 | 0.087777402 |
Ribosome | 0.238028817 | 0.241641621 | 0.010304191 | 0.02009132 | 0.238028817 |
Cytosol | 0.417439425 | 0.370390729 | 0.044421524 | 0.080425189 | 0.417439425 |
Exosome | 0.022304697 | 0.023739093 | 0.005925556 | 0.023492704 | 0.022304697 |
Clinical significance of the LCMT1-AS2 regulatory axis in COAD
To clarify the clinical significance of the ten genes obtained from the above analysis in COAD, we adopted multi-omics methods to explore them in subsequent studies. First, we evaluated the expression of the genes in the tumor tissues of patients with COAD. MiR-4676-5p was significantly downregulated in the COAD tissues compared with adjacent tissues, whereas miR-4446-5p expression did not change significantly. MiR-454-3p, miR-506-3p, and miR-4306 exhibited extremely low levels of expression in eight adjacent non-cancerous tissues, but were present in higher levels in colon cancer tissues. LCMT1-AS2, RPS6KA5, PRRT2, ABCB5, and BAHD1 were significantly underexpressed in the COAD tissues in contrast to their expression in normal colon tissues (Figure 4A).
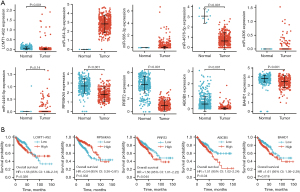
Cox regression analysis then revealed that patients with COAD in the high LCMT1-AS2, ABCB5, PRRT2, or BAHD1 group had poorer OS than those in the low-expression groups, whereas the RPS6KA5 low-expression group had poorer OS and miR-454-3p and miR-4676-5p expression levels were independent of OS (Figure 4B). Owing to the extremely low expression of more than half of the specimens, the correlations of miR-506-3p, miR-4306, and miR-4446-5p with OS was not analyzed.
Correlation analysis between gene expression levels and clinicopathological parameters was subsequently performed. Wilcoxon rank-sum test analysis showed that miR-454-3p expression was significantly associated with lymphatic invasion and perineural invasion and independent of gender, T stage, N stage, distant metastasis, pathological stage, and serum CEA expression level. MiR-4676-5p was associated with distant metastasis and lymphatic invasion and was not associated with other clinical factors. RPS6KA5 expression was related to tumor-node-metastasis (TNM) stage, pathological stage, and lymphatic invasion. PRRT2 expression was significantly associated with N staging and pathological grading (Figure S4). LCMT1-AS2, ABCB5, or BAHD1 expression level was not correlated with clinical pathological factors.
A significant positive correlation between LCMT1-AS2 and RPS6KA5 was found (Figure 3B; Spearman r=0.58, P<0.05), and both were correlated with the OS of patients with COAD. Alternatively, we detected significantly high expression of miR-454-3p and low expression of RPS6KA5 in COAD tissues, and both were related to clinicopathological factors. Therefore, the LCMT1-AS2/miR-454-3p/RPS6KA5 axis was finally established. To understand the mechanism of action of this regulatory axis in colon cancer, we performed a detailed analysis of RPS6KA5.
Expression validation of RPS6KA5 and enrichment analysis
Independent cohort data (GSE10950: 24 pairs of COAD samples and paracancerous samples) presented within GEO database provided evidence of the downregulation of RPS6KA5 expression in cancer tissues (Figure 5A). Similarly, the representative images of immunohistochemical staining from the HPA database suggested that RPS6KA5 had low expression level in the colon cancer tissues (Figure 5B). Moreover, the expression levels of RPS6KA5 in various malignant tumor cell lines (Figure S5) and CRC cell lines were queried in CCLE database (Figure S6).
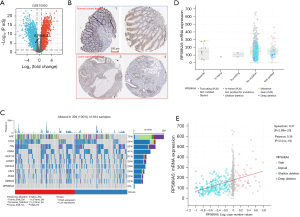
Figure 5C shows the somatic mutation landscape in TCGA-COAD cohort, with a mutation rate of about 2% in RPS6KA5 and a higher frequency of somatic mutations in the RPS6KA5 high-expression group. The mutation types, structural variation, and copy number alterations (CNAs) of RPS6KA5 for 594 CRC patient samples from TCGA database were queried in the cBioPortal database (Figure 5D,5E). A significant positive association was found between the copy number value of RPS6KA5 and mRNA expression (Spearman r=0.37; P<0.05).
Differential expression analysis was performed on the RPS6KA5 high-expression and low-expression groups [|log(FC)| >1.5; Figure 6A,6B]. Subsequently, 170 upregulated genes and 29 downregulated genes were subjected to enrichment analysis (Figure 6C). The two gene sets were mostly enriched in the PI3K-Akt signaling pathway.
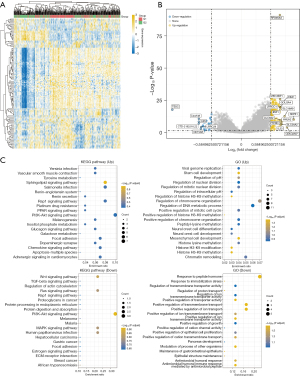
Analysis of functional mechanism related to RPS6KA5
Patients with COAD were evenly divided into four groups according to their RPS6KA5 expression levels (Figure 7A). The top 25% group (RPS6KA5high25%) had the longest median OS (7.1 years), and the bottom 25% group (RPS6KA5low25%) had the shortest median OS (5 years; Figure 7B). The areas under the receiver operating characteristic (ROC) curve (AUCs) for predicting 1-, 3- and 5-year survival probability were 0.542, 0.584, and 0.644, respectively (Figure 7C).
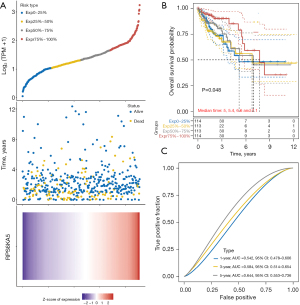
Ferroptosis
Ferroptosis is an iron-mediated regulatory cell death and has the dual role of promoting and inhibiting tumors during tumorigenesis. It affects the efficacy of chemotherapy, radiotherapy, and immunotherapy (30). We compared the expression levels of 24 FRGs in the RPS6KA5high25% and RPS6KA5low25% groups to determine whether RPS6KA5 is associated with ferroptosis. Wilcoxon test suggested that the expression of 16 FRGs differed significantly between the two groups (Figure 8A), indicating that RPS6KA5 is involved in regulating ferroptosis in colon cancer.
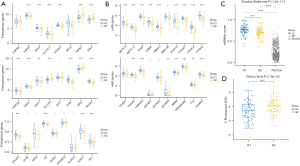
M6A methylation
M6A methylation is one of the most common epigenetic modifications in mRNA. Enzymes involved in m6A modification can cause various diseases, including tumors (31). Herein, we found that compared with the RPS6KA5low25% group, the RPS6KA5high25% group had 16 genes correlated with the three types of regulatory enzymes (m6A writers, readers, and erasers) with high expression levels. Only RBM15B, YTHDF1, IGF2BP1, and IGF2BP2 were not expressed differently between the two groups (Figure 8B).
Tumor stemness
Cancer progression is accompanied by the gradual loss of tumor cell differentiation phenotype and the acquisition of stem cell-like characteristics (24), and the stemness of cancer cells is closely related to tumor proliferation and metastasis and drug resistance (31). Based on the one-class logistic regression (OCLR) algorithm (24), the degree of stemness of cancer samples can be assessed by calculating their stemness index. We found that the mRNAsi of COAD tissues was markedly increased compared with that of normal colon tissues, and the mRNAsi of the RPS6KA5high25% group was significantly higher than that of the RPS6KA5low25% group (Figure 8C).
Chemotherapy
5-FU is one of the most commonly used chemotherapy drugs for colon cancer. Using the GDSC database, we determined whether RPS6KA5 expression levels affected the response of patients with colon cancer to drug therapy with 5-FU. The IC50 of 5-FU in each sample was calculated, and the IC50 value distributions of the RPS6KA5high25% and RPS6KA5low25% groups were compared. We found that the RPS6KA5low25% group had significantly higher IC50 values that the RPS6KA5high25% group (Figure 8D). This result indicated that the RPS6KA5low25% group responded more poorly to 5-FU treatment.
Immune infiltration analysis
Based on the TIMER database, ‘Gene’ module analysis suggested that RPS6KA5 expression was negatively correlated with tumor purity. After purity correction, RPS6KA5 expression was found to be positively correlated with the infiltration abundance of B cells, CD8+ T cells, CD4+ T cells, macrophages, neutrophils, and dendritic cells in COAD (Figure 9A). ‘sCNA’ module analysis showed the difference in the immune cell infiltration ESTIMATE level among tumors with different CNA status of RPS6KA5 in COAD. As shown in Figure 9B, the arm-level deletion of RPS6KA5 was largely responsible for the infiltration levels of various immune cells types. The stromal, immune, and ESTIMATE scores of tumor samples were calculated. Correlation analysis showed that all three were positively correlated with the expression levels of RPS6KA5 (Figure 9C), suggesting that the higher proportion of non-tumor cells increased with the expression level of RPS6KA5. This effect lowered tumor purity in COAD tissues.
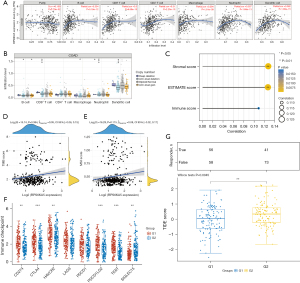
Relationship between immunotherapy and RPS6KA5 expression
Immune checkpoint inhibitor (ICI) therapy has emerged as an important therapeutic method for patients with colon cancer. Currently, programmed cell death protein 1/programmed death ligand 1 (PD-1/PD-L1) expression status, tumor mutational burden (TMB), and microsatellite instability (MSI) as effective predictive markers for ICI treatment have been extensively studied. We investigated whether RPS6KA5 expression affected the efficacy of immunotherapy in patients with COAD from multiple aspects. First, our analysis revealed that RPS6KA5 expression had no correlation with TMB (Figure 9D) and MSI (Figure 9E). Second, given that the mechanism of immune checkpoint molecules expressed on immune cells facilitate tumor cell immune escape by inhibiting the function of immune cells (32), we performed corresponding analysis. The study revealed that the expression levels of the immune checkpoint molecules of CD274 (PD-L1), CTLA4, HAVCR2 (Tim-3), PDCD1LG2 (PD-L2), and TIGIT were significantly increased in the RPS6KA5high25% group, whereas SIGLEC15 expression in RPS6KA5low25% had a higher increase rate and the expression levels of LAG3 (CD223) and PDCD1 (PD-1) had no difference between the two groups (Figure 9F). Finally, the tumor immune dysfunction and exclusion (TIDE) calculation method (33) was used in assessing the likelihood of tumor immune escape and predicting response to ICI treatment. Compared with the RPS6KA5low25% group, the RPS6KA5high25% group had significantly higher TIDE scores (P=0.0045; Figure 9G), indicating that the RPS6KA5high25% group had a higher possibility of immune escape and a poorer response to ICI treatment.
Discussion
CEACAM5 encodes CEA, a clinical biomarker for CRC, and contributes to tumorigenesis as a cell adhesion molecule (5,6). Our research identified a significant elevation in CEACAM5 expression within CRC tissues, which was found to be correlated with the prognostic outcomes of CRC. Increasing experimental evidence supports that the ceRNA regulatory network plays a role in diverse cancer types (34,35). However, the specific mechanisms and complex networks of the ceRNA regulatory pattern in COAD remain unclear. Given the pivotal role of CEACAM5 in COAD, we proceeded to construct the lncRNA-miRNA-mRNA triple networks correlated with CEACAM5 and establish a novel regulatory axis associated with prognosis in patients with COAD. Then, we explored the mechanisms and functions of this regulatory axis from a multi-omics perspective and attempted to corroborate the ceRNA regulatory pattern of lncRNA involvement in COAD.
We set up a ceRNA network of 18 lncRNAs, 177 miRNAs, and 25 mRNAs associated with CEACAM5 was constructed. LCMT1-AS2 is located primarily in the cytoplasm, thereby meeting the prerequisites for lncRNA-miRNA interactions. In the prediction results, the ceRNA network regulated by LCMT1-AS2 was largest and most complex. LCMT1-AS2 was significantly positively correlated with RPS6KA5, and the key LCMT1-AS2/miR-454-3p/RPS6KA5 regulatory axis was finally established after further correlation analysis of clinicopathological factors and survival analysis. In accordance with the ceRNA hypothesis, we speculated that LCMT1-AS2 may competitively bind miR-454-3p to miRNA response elements, thereby affecting RPS6KA5 expression. In our study, the functions and mechanisms of RPS6KA5 were analyzed using the bioinformatic method, which indirectly reflected the mechanism of action of the regulatory axis of LCMT1-AS2/RPS6KA5.
By searching these three genes in PubMed, we found that the roles of miR-454-3p and RPS6KA5 in cancer have been studied, whereas the mechanism of action of LCMT1-AS2 is unknown and has not been explored by relevant research. MiR-454-3p can play a role as an oncogene in cervical cancer, glioma, stomach cancer, pancreatic cancer, malignant melanoma, and other solid tumors (36-40). Existing research RPS6KA5, also known as MSK1, MSPK1, or RLPK, plays a role in CRC. Bile acid upregulates MUC2 transcription and contributes to carcinogenesis within colorectal tissues by activating the EGFR/PKC/Ras/Raf-1/MEK1/ERK/CREB, PI3/Akt/IkappaB/NF-kappaB, and p38/MSK1/CREB pathways (41). Vitamin D receptor acts as a transcription factor and a nongenomic activator of p38MAPK-MSK1 and RhoA-ROCK, assisting 1,25(OH)2D3 in inhibiting proliferation and promoting the differentiation of colon cancer cells (42). Our study found that RPS6KA5 expression was downregulated in COAD tissues. Its copy number was significantly positively correlated with gene expression level, and low expression predicted poor OS. The abnormal expression of RPS6KA5 may play a role in colon cancer through the PI3K-Akt signaling pathway. Additionally, we found that most FRGs were highly expressed in the RPS6KA5high25% group, and this result indicated that the downregulation of RPS6KA5 affects ferroptosis and inhibits the progression of colon cancer. This result is in line with the observations presented by Xie et al. that ferroptosis suppresses the growth of colon cancer (43). Several studies have shown that the abnormal methylation levels of m6A are associated with stem cell differentiation (44), immune response (45), immune cell infiltration (46), tumorigenesis, and tumor cell proliferation and metastasis (47,48). The present study demonstrated that a significant decrease in RPS6KA5 expression may lead to a decrease in the m6A methylation modification and demodification/modification levels. It has been proven that the increased expression of YTHDF2, RBMX, and RBM15 was correlated with the activation of the PI3K/Akt/mTOR signaling pathways (49). Combined with the results of our KEGG enrichment analysis, the relationship between RPS6KA5 expression dysregulation and the PI3K-Akt signaling pathway was further confirmed. Moreover, the mRNAsi index of colon cancer tissues was significantly increased compared with that of normal colon tissues and was higher in the RPS6KA5high25% group than that in the RPS6KA5low25% group. The RPS6KA5low25% group responded more poorly to 5-FU therapy possibly because of enhanced resistance to 5-FU caused by significantly decreased RPS6KA5 expression.
RPS6KA5 may affect the immune infiltration of COAD. The downregulation of RPS6KA5 expression was accompanied by a significant decline in the infiltrating abundance of B cells, CD8+ T cells, CD4+ T cells, macrophages, neutrophils, and dendritic cells, decrease in the proportion of non-tumor cells, and increase in tumor purity in the colon cancer tissues. RPS6KA5 expression was not correlated with TMB and MSI. However, correlation analysis on immune checkpoint molecules and TIDE algorithm showed similar results. That is, the RPS6KA5high25% group had a higher likelihood of immune evasion and benefit less from ICI treatment.
PD-L1 and PD-1 expression levels weakly predict the responses of CRC patients to ICI treatment and are not commonly used as clinical predictors; meanwhile, patients with high microsatellite instability (MSI-H)/deficient mismatch repair (dMMR) CRC are sensitive to ICI treatment (50). In summary, significantly decreased RPS6KA5 expression leads to decreased immune-cell infiltration abundance and weakened immune evasion. However, whether this change affects colon cancer progression and response to ICI treatment requires further study.
Conclusions
An LCMT1-AS2/miR-454-3p/RPS6KA5 axis associated with COAD prognosis was established, which may be an important prognostic factor predicting the clinical outcome of COAD and may contribute to our understanding of lncRNA-miRNA-mRNA interactions. There are several limitations of this study that are worth discussing. First, our study is based on bioinformatics analysis, so the binding affinity of lncRNA-miRNA and miRNA-mRNA pairs obtained from the database should be verified experimentally. Second, more experimental studies on the mechanisms and functions of the LCMT1-AS2/miR-454-3p/RPS6KA5 axis in COAD are imperative. Generally, our findings suggest that the LCMT1-AS2/RPS6KA5 axis is a promising new candidate for potential prognostic biomarker and therapeutic target for COAD, which can be applied to facilitate individualized treatment strategies.
Acknowledgments
We sincerely acknowledge all the online databases for the availability of the data.
Funding: None.
Footnote
Reporting Checklist: The authors have completed the TRIPOD reporting checklist. Available at https://jgo.amegroups.com/article/view/10.21037/jgo-24-43/rc
Peer Review File: Available at https://jgo.amegroups.com/article/view/10.21037/jgo-24-43/prf
Conflicts of Interest: All authors have completed the ICMJE uniform disclosure form (available at https://jgo.amegroups.com/article/view/10.21037/jgo-24-43/coif). The authors have no conflicts of interest to declare.
Ethical Statement: The authors are accountable for all aspects of the work in ensuring that questions related to the accuracy or integrity of any part of the work are appropriately investigated and resolved. The study was conducted in accordance with the Declaration of Helsinki (as revised in 2013).
Open Access Statement: This is an Open Access article distributed in accordance with the Creative Commons Attribution-NonCommercial-NoDerivs 4.0 International License (CC BY-NC-ND 4.0), which permits the non-commercial replication and distribution of the article with the strict proviso that no changes or edits are made and the original work is properly cited (including links to both the formal publication through the relevant DOI and the license). See: https://creativecommons.org/licenses/by-nc-nd/4.0/.
References
- Sung H, Ferlay J, Siegel RL, et al. Global Cancer Statistics 2020: GLOBOCAN Estimates of Incidence and Mortality Worldwide for 36 Cancers in 185 Countries. CA Cancer J Clin 2021;71:209-49. [Crossref] [PubMed]
- Hao C, Zhang G, Zhang L. Serum CEA levels in 49 different types of cancer and noncancer diseases. Prog Mol Biol Transl Sci 2019;162:213-27. [Crossref] [PubMed]
- Hatakeyama K, Wakabayashi-Nakao K, Ohshima K, et al. Novel protein isoforms of carcinoembryonic antigen are secreted from pancreatic, gastric and colorectal cancer cells. BMC Res Notes 2013;6:381. [Crossref] [PubMed]
- Nicholson BD, Shinkins B, Pathiraja I, et al. Blood CEA levels for detecting recurrent colorectal cancer. Cochrane Database Syst Rev 2015;2015:CD011134. [Crossref] [PubMed]
- Shinkins B, Nicholson BD, Primrose J, et al. The diagnostic accuracy of a single CEA blood test in detecting colorectal cancer recurrence: Results from the FACS trial. PLoS One 2017;12:e0171810. [Crossref] [PubMed]
- Saeland E, Belo AI, Mongera S, et al. Differential glycosylation of MUC1 and CEACAM5 between normal mucosa and tumour tissue of colon cancer patients. Int J Cancer 2012;131:117-28. [Crossref] [PubMed]
- van Gisbergen KP, Aarnoudse CA, Meijer GA, et al. Dendritic cells recognize tumor-specific glycosylation of carcinoembryonic antigen on colorectal cancer cells through dendritic cell-specific intercellular adhesion molecule-3-grabbing nonintegrin. Cancer Res 2005;65:5935-44. [Crossref] [PubMed]
- Gu S, Zaidi S, Hassan MI, et al. Mutated CEACAMs Disrupt Transforming Growth Factor Beta Signaling and Alter the Intestinal Microbiome to Promote Colorectal Carcinogenesis. Gastroenterology 2020;158:238-52. [Crossref] [PubMed]
- Kim T, Croce CM. Long noncoding RNAs: Undeciphered cellular codes encrypting keys of colorectal cancer pathogenesis. Cancer Lett 2018;417:89-95. [Crossref] [PubMed]
- Salmena L, Poliseno L, Tay Y, et al. A ceRNA hypothesis: the Rosetta Stone of a hidden RNA language? Cell 2011;146:353-8. [Crossref] [PubMed]
- Thomson DW, Dinger ME. Endogenous microRNA sponges: evidence and controversy. Nat Rev Genet 2016;17:272-83. [Crossref] [PubMed]
- Wang P, Ning S, Zhang Y, et al. Identification of lncRNA-associated competing triplets reveals global patterns and prognostic markers for cancer. Nucleic Acids Res 2015;43:3478-89. [Crossref] [PubMed]
- Poliseno L, Salmena L, Zhang J, et al. A coding-independent function of gene and pseudogene mRNAs regulates tumour biology. Nature 2010;465:1033-8. [Crossref] [PubMed]
- Lin L, Zeng X, Liang S, et al. Construction of a co-expression network and prediction of metastasis markers in colorectal cancer patients with liver metastasis. J Gastrointest Oncol 2022;13:2426-38. [Crossref] [PubMed]
- Mayakonda A, Lin DC, Assenov Y, et al. Maftools: efficient and comprehensive analysis of somatic variants in cancer. Genome Res 2018;28:1747-56. [Crossref] [PubMed]
- Love MI, Huber W, Anders S. Moderated estimation of fold change and dispersion for RNA-seq data with DESeq2. Genome Biol 2014;15:550. [Crossref] [PubMed]
- Sticht C, De La Torre C, Parveen A, et al. miRWalk: An online resource for prediction of microRNA binding sites. PLoS One 2018;13:e0206239. [Crossref] [PubMed]
- Wang X. Improving microRNA target prediction by modeling with unambiguously identified microRNA-target pairs from CLIP-ligation studies. Bioinformatics 2016;32:1316-22. [Crossref] [PubMed]
- Wong N, Wang X. miRDB: an online resource for microRNA target prediction and functional annotations. Nucleic Acids Res 2015;43:D146-52. [Crossref] [PubMed]
- Chou CH, Shrestha S, Yang CD, et al. miRTarBase update 2018: a resource for experimentally validated microRNA-target interactions. Nucleic Acids Res 2018;46:D296-302. [Crossref] [PubMed]
- Yu G, Wang LG, Han Y, et al. clusterProfiler: an R package for comparing biological themes among gene clusters. OMICS 2012;16:284-7. [Crossref] [PubMed]
- Kang R, Kroemer G, Tang D. The tumor suppressor protein p53 and the ferroptosis network. Free Radic Biol Med 2019;133:162-8. [Crossref] [PubMed]
- Liu Z, Zhao Q, Zuo ZX, et al. Systematic Analysis of the Aberrances and Functional Implications of Ferroptosis in Cancer. iScience 2020;23:101302. [Crossref] [PubMed]
- Malta TM, Sokolov A, Gentles AJ, et al. Machine Learning Identifies Stemness Features Associated with Oncogenic Dedifferentiation. Cell 2018;173:338-354.e15. [Crossref] [PubMed]
- Wang Z, Wang Y, Yang T, et al. Machine learning revealed stemness features and a novel stemness-based classification with appealing implications in discriminating the prognosis, immunotherapy and temozolomide responses of 906 glioblastoma patients. Brief Bioinform 2021;22:bbab032. [Crossref] [PubMed]
- Yoshihara K, Shahmoradgoli M, Martínez E, et al. Inferring tumour purity and stromal and immune cell admixture from expression data. Nat Commun 2013;4:2612. [Crossref] [PubMed]
- Laubert T, Bente V, Freitag-Wolf S, et al. Aneuploidy and elevated CEA indicate an increased risk for metachronous metastasis in colorectal cancer. Int J Colorectal Dis 2013;28:767-75. [Crossref] [PubMed]
- Zhai H, Huang J, Yang C, et al. Serum CEA and CA19-9 Levels are Associated with the Presence and Severity of Colorectal Neoplasia. Clin Lab 2018;64:351-6. [Crossref] [PubMed]
- Liu J, Lichtenberg T, Hoadley KA, et al. An Integrated TCGA Pan-Cancer Clinical Data Resource to Drive High-Quality Survival Outcome Analytics. Cell 2018;173:400-416.e11. [Crossref] [PubMed]
- Chen X, Kang R, Kroemer G, et al. Broadening horizons: the role of ferroptosis in cancer. Nat Rev Clin Oncol 2021;18:280-96. [Crossref] [PubMed]
- Fu Y, Dominissini D, Rechavi G, et al. Gene expression regulation mediated through reversible m6A RNA methylation. Nat Rev Genet 2014;15:293-306. [Crossref] [PubMed]
- Topalian SL. Targeting Immune Checkpoints in Cancer Therapy. JAMA 2017;318:1647-8. [Crossref] [PubMed]
- Hansen TB, Jensen TI, Clausen BH, et al. Natural RNA circles function as efficient microRNA sponges. Nature 2013;495:384-8. [Crossref] [PubMed]
- Wu C, Hou X, Li S, et al. Identification of a potential competing endogenous RNA (ceRNA) network in gastric adenocarcinoma. J Gastrointest Oncol 2023;14:1019-36. [Crossref] [PubMed]
- Zhang X, Han X, Zuo P, et al. CEACAM5 stimulates the progression of non-small-cell lung cancer by promoting cell proliferation and migration. J Int Med Res 2020;48:300060520959478. [Crossref] [PubMed]
- Song Y, Guo Q, Gao S, et al. miR-454-3p promotes proliferation and induces apoptosis in human cervical cancer cells by targeting TRIM3. Biochem Biophys Res Commun 2019;516:872-9. [Crossref] [PubMed]
- Zuo J, Yu H, Xie P, et al. miR-454-3p exerts tumor-suppressive functions by down-regulation of NFATc2 in glioblastoma. Gene 2019;710:233-9. [Crossref] [PubMed]
- Jiang D, Li H, Xiang H, et al. Long Chain Non-Coding RNA (lncRNA) HOTAIR Knockdown Increases miR-454-3p to Suppress Gastric Cancer Growth by Targeting STAT3/Cyclin D1. Med Sci Monit 2019;25:1537-48. [Crossref] [PubMed]
- Fan Y, Xu LL, Shi CY, et al. MicroRNA-454 regulates stromal cell derived factor-1 in the control of the growth of pancreatic ductal adenocarcinoma. Sci Rep 2016;6:22793. [Crossref] [PubMed]
- Sun L, Wang Q, Gao X, et al. MicroRNA-454 functions as an oncogene by regulating PTEN in uveal melanoma. FEBS Lett 2015;589:2791-6. [Crossref] [PubMed]
- Lee HY, Crawley S, Hokari R, et al. Bile acid regulates MUC2 transcription in colon cancer cells via positive EGFR/PKC/Ras/ERK/CREB, PI3K/Akt/IkappaB/NF-kappaB and p38/MSK1/CREB pathways and negative JNK/c-Jun/AP-1 pathway. Int J Oncol 2010;36:941-53. [Crossref] [PubMed]
- Ordóñez-Morán P, Larriba MJ, Pálmer HG, et al. RhoA-ROCK and p38MAPK-MSK1 mediate vitamin D effects on gene expression, phenotype, and Wnt pathway in colon cancer cells. J Cell Biol 2008;183:697-710. [Crossref] [PubMed]
- Xie Y, Zhu S, Song X, et al. The Tumor Suppressor p53 Limits Ferroptosis by Blocking DPP4 Activity. Cell Rep 2017;20:1692-704. [Crossref] [PubMed]
- Wang S, Sun C, Li J, et al. Roles of RNA methylation by means of N(6)-methyladenosine (m(6)A) in human cancers. Cancer Lett 2017;408:112-20. [Crossref] [PubMed]
- Han D, Liu J, Chen C, et al. Anti-tumour immunity controlled through mRNA m(6)A methylation and YTHDF1 in dendritic cells. Nature 2019;566:270-4. [Crossref] [PubMed]
- Han S, Qi J, Fang K, et al. Characterization of m6A regulator-mediated methylation modification patterns and tumor microenvironment infiltration in acute myeloid leukemia. Cancer Med 2022;11:1413-26. [Crossref] [PubMed]
- Cui Q, Shi H, Ye P, et al. m(6)A RNA Methylation Regulates the Self-Renewal and Tumorigenesis of Glioblastoma Stem Cells. Cell Rep 2017;18:2622-34. [Crossref] [PubMed]
- Chen M, Wei L, Law CT, et al. RNA N6-methyladenosine methyltransferase-like 3 promotes liver cancer progression through YTHDF2-dependent posttranscriptional silencing of SOCS2. Hepatology 2018;67:2254-70. [Crossref] [PubMed]
- Li Y, Xiao J, Bai J, et al. Molecular characterization and clinical relevance of m(6)A regulators across 33 cancer types. Mol Cancer 2019;18:137. [Crossref] [PubMed]
- Oliveira AF, Bretes L, Furtado I. Review of PD-1/PD-L1 Inhibitors in Metastatic dMMR/MSI-H Colorectal Cancer. Front Oncol 2019;9:396. [Crossref] [PubMed]