A prognostic nomogram integrating carcinoembryonic antigen levels for predicting overall survival in elderly patients with stage II–III colorectal cancer
Highlight box
Key findings
• We constructed a prediction nomogram for stage II–III elderly colorectal cancer (CRC) patients which can accurately predict patient survival.
What is known and what is new?
• Surgery and adjuvant therapy remain important treatments for patients with stage II–III CRC, however, they have not been adequately discussed in the elderly population.
• Our study added carcinoembryonic antigen levels and lymph node dissection to construct a nomogram, enabling patients to achieve a greater net benefit in treatment decisions than using the traditional tumor-node-metastasis staging system.
What is the implication, and what should change now?
• We constructed a survival prediction nomogram for elderly patients with stage II–III CRC firstly, which facilitates clinicians to accurately identify high-risk patients and adopt more aggressive treatment strategies. Clinicians need to pay more attention to the diagnosis and treatment of elderly CRC patients.
Introduction
Colorectal cancer (CRC) is the third most common cancer in the world and the second leading cause of cancer-related deaths globally (1). In 2020, there were more than 1.9 million new CRC cases and more than 930,000 deaths worldwide (1). It is estimated that in 2040, there will be 3.2 million new cases of CRC globally and about 1.6 million deaths due to CRC (2). China and the United States will be the countries with the largest number of new CRC cases in the next 20 years (3). Although CRC has good treatment prospects, CRC still poses a heavy economic burden and a huge healthcare challenge for both developed and developing countries (4-6). Reduced physical activity, excess weight, poor diet, excessive alcohol consumption, and smoking are considered variable risk factors for CRC (2). Epidemiological studies have shown that males and advancing age have been strongly associated with the incidence of CRC (2,7,8). With the aging of the population, colorectal surgeons will have to deal with older patients in the future than they do today (9). It is estimated that in 2023, elderly patients (age ≥65 years) will account for approximately 56% of new CRC patients in the United States (10). Thus, more attention should be paid to the diagnosis and prognosis of the elderly CRC patient group.
For traditional stage II–III CRC patients, the standard treatment is radical resection and adjuvant chemoradiotherapy (11). However, elderly CRC patients are a special category because they are older, in poorer health, have more underlying diseases and do not tolerate surgery and adjuvant chemoradiotherapy as well as younger CRC patients (12,13). At present, the prognostic analysis and risk factor analysis of elderly CRC patients are still lacking. The traditional tumor-node-metastasis (TNM) staging system is widely used for the prognostic prediction of CRC patients (14). However, there is growing evidence that the introduction of other observational indicators based on the TNM staging system performs better than using the TNM staging system alone to predict the patient’s prognosis (15,16).
In this study, we propose to construct a survival prediction nomogram for elderly CRC patients with stage II–III by combining the pre-treatment carcinoembryonic antigen (CEA) level and the treatments received by the patients, so that clinicians can better assess the prognosis of the patients, quickly identify high-risk patients, and more actively adopt treatment strategies to ultimately improve the survival of the patients. We present this article in accordance with the TRIPOD reporting checklist (available at https://jgo.amegroups.com/article/view/10.21037/jgo-23-863/rc).
Methods
Data source and retrieval criteria
All patient data for this study were obtained from the Surveillance, Epidemiology, and End Results (SEER) database (https://seer.cancer.gov/) (SEER*Stat software, version 8.4.1.2). The database is an open-source database that allows public access and complies with applicable laws and regulations. The study was conducted in accordance with the Declaration of Helsinki (as revised in 2013). No additional ethics committee approval was required for this study. We used SEER*Stat software, version 8.4.1.2 [Incidence-SEER Research Data12 Registers Nov 2022 Sub (1992–2020)] to retrieve patients with primary colorectal malignant tumors registered in the SEER database from 2010 to 2015. Included indicators include: (I) primary colorectal malignant tumors; (II) age ≥65 years; (III) chemotherapy and carcinoembryonic antigen (CEA) pretreatment record; (IV) surgery perform record; (V) radiation record; (VI) chemotherapy record. Exclusion criteria included: the primary location is the appendix; patients with TNM stage I, II not otherwise specified (NOS), III NOS and IV [American Joint Committee on Cancer (AJCC) TNM stage, 8th ed] (17); exclude number of regional lymph nodes removed unknown; T stage and N stage NOS. Finally, 3,016 stage II–III elderly CRC patients were included, and all patients were randomly split into training and validation sets in a 7:3 ratio. The specific flow chart is shown in Figure 1.
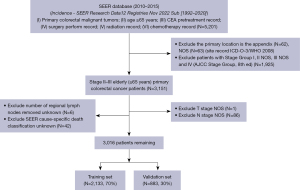
Feature selection and construction of the nomogram
All potential prognostic factors were first screened in the training set using univariate Cox regression analyses and clinical features with P<0.05 were selected. Candidate factors were then further screened using the least absolute shrinkage and selection operator (LASSO) regression algorithm, where the shrinkage and selection of variables were achieved through a logistic regression model with LASSO penalties, and λ-values were determined through iterative cross-validation (18). Significant variables screened by LASSO were finally analyzed using multivariate Cox proportional hazards analyses, and statistically significant (P<0.05) variables were used to further construct a patient 3- and 5-year overall survival (OS) prediction nomogram.
Validation and performance of nomogram
The area under the curve (AUC) value and consistency index (C-index) were used to evaluate the discrimination ability of nomogram. The AUC value was established based on the receiver operating characteristic (ROC) curve, which was used to assess the accuracy of the nomogram. C-index was calculated based on the Cox regression model method, which was used to assess the discriminative ability of the nomogram, and the C-index was between 0.5 and 1.0, which indicated that the nomogram had good discriminative ability. The k-fold cross validation (k=10) was used for further internal validation of the model. We further assessed the goodness-of-fit of the nomogram by using calibration plots, using validation sets for external validation of the model. The C-index, decision curve analysis (DCA), Akaike information criterion (AIC), and Bayesian information criterion (BIC) were used to compare the predictive ability between the nomogram and TNM stage system. Based on the patient survival prediction nomogram constructed from the training set, we calculated risk scores for all patients, and determined the optimal cut point using the maximally selected rank statistics from the ‘maxstat’ R package. All patients were divided into high and low risk groups based on the optimal cut point value and the difference in survival between the two groups was compared using Kaplan-Meier survival analysis (log-rank test).
Statistical analysis
The Chi-square test was used to compare categorical variables. Survival curves were performed by Kaplan-Meier method and compared by log-rank test (19). All statistical analyses and visualization of results were performed using R-4.3.1 (https://www.r-project.org/) and R studio (https://posit.co/products/open-source/rstudio/). P<0.05 was considered statistically significant.
Results
Patient baseline characteristics
A total of 3,016 elderly (age ≥65 years) patients with stage II–III CRC were included in this study. Among them, 1,509 (50%) were female and 1,507 (50%) were male. The most common location of the tumor was sigmoid colon in about 20.9% (N=629), followed by ascending colon (18.3%, N=553) and cecum (17.8%, N=538). In the patient population in this study, stage IIA patients were the most numerous with a percentage of approximately 43.2% (N=1,304), followed by stage IIIB with approximately 34.8% (N=1,050). In T-stage, T3 was the most prevalent at about 76.4% (N=2,303), and in N-stage, N0 accounted for about 49.6%, followed by N1a (16.4%, N=494) N1b (15.8%, N=476). About 96.8% (N=2,919) of patients received surgical treatment and 92.7% of patients were with 4 or more lymph node dissections. 90.1% (N=2,717) of patients did not receive radiotherapy treatment, and only 37.4% (N=1,129) of patients received chemotherapy. About half of patients (50.1%) had increased pre-treatment CEA levels. All patients were randomly divided into training and validation sets in a 7:3 ratio, with no statistically significant difference between the two groups (P>0.05). The baseline characteristics of the patients are shown in Table 1.
Table 1
Characteristics | Total (N=3,016), n (%) | Training set (N=2,133), n (%) | Validation set (N=883), n (%) | P value |
---|---|---|---|---|
Age (years) | 0.713 | |||
65–69 | 752 (24.9) | 522 (24.5) | 230 (26.0) | |
70–74 | 663 (22.0) | 467 (21.9) | 196 (22.2) | |
75–79 | 586 (19.4) | 425 (19.9) | 161 (18.2) | |
80–84 | 544 (18.0) | 380 (17.8) | 164 (18.6) | |
85+ | 471 (15.6) | 339 (15.9) | 132 (14.9) | |
Sex | 0.591 | |||
Female | 1,509 (50.0) | 1,060 (49.7) | 449 (50.8) | |
Male | 1,507 (50.0) | 1,073 (50.3) | 434 (49.2) | |
Marital status | 0.702 | |||
Divorced | 260 (8.6) | 185 (8.7) | 75 (8.5) | |
Married | 1,470 (48.7) | 1,021 (47.9) | 449 (50.8) | |
Separated | 32 (1.1) | 23 (1.1) | 9 (1.0) | |
Unknown | 150 (5.0) | 104 (4.9) | 46 (5.2) | |
Unmarried | 409 (13.6) | 298 (14.0) | 111 (12.6) | |
Widowed | 695 (23.0) | 502 (23.5) | 193 (21.9) | |
Race | 0.764 | |||
Asian or Pacific Islander | 1,914 (63.5) | 1,345 (63.1) | 569 (64.4) | |
Black | 920 (30.5) | 657 (30.8) | 263 (29.8) | |
Other | 182 (6.0) | 131 (6.1) | 51 (5.8) | |
Tumor site | 0.401 | |||
Ascending colon | 553 (18.3) | 407 (19.1) | 146 (16.5) | |
Cecum | 538 (17.8) | 378 (17.7) | 160 (18.1) | |
Descending colon | 166 (5.5) | 118 (5.5) | 48 (5.4) | |
Hepatic flexure | 131 (4.3) | 99 (4.6) | 32 (3.6) | |
Rectosigmoid junction | 221 (7.3) | 162 (7.6) | 59 (6.7) | |
Rectum | 401 (13.3) | 273 (12.8) | 128 (14.5) | |
Sigmoid colon | 629 (20.9) | 428 (20.1) | 201 (22.8) | |
Splenic flexure | 108 (3.6) | 79 (3.7) | 29 (3.3) | |
Transverse colon | 269 (8.9) | 189 (8.9) | 80 (9.1) | |
TNM stage | 0.287 | |||
IIA | 1,304 (43.2) | 901 (42.2) | 403 (45.6) | |
IIB | 94 (3.1) | 70 (3.3) | 24 (2.7) | |
IIC | 97 (3.2) | 74 (3.5) | 23 (2.6) | |
IIIA | 166 (5.5) | 114 (5.3) | 52 (5.9) | |
IIIB | 1,050 (34.8) | 747 (35.0) | 303 (34.3) | |
IIIC | 305 (10.1) | 227 (10.6) | 78 (8.8) | |
T stage | 0.107 | |||
T1 | 63 (2.1) | 46 (2.2) | 17 (1.9) | |
T2 | 137 (4.5) | 96 (4.5) | 41 (4.6) | |
T3 | 2,303 (76.4) | 1,610 (75.5) | 693 (78.5) | |
T4a | 325 (10.8) | 232 (10.9) | 93 (10.5) | |
T4b | 188 (6.2) | 149 (7.0) | 39 (4.4) | |
N stage | 0.885 | |||
N0 | 1,495 (49.6) | 1,045 (49.0) | 450 (51.0) | |
N1a | 494 (16.4) | 353 (16.5) | 141 (16.0) | |
N1b | 476 (15.8) | 336 (15.8) | 140 (15.9) | |
N1c | 70 (2.3) | 51 (2.4) | 19 (2.2) | |
N2a | 296 (9.8) | 217 (10.2) | 79 (8.9) | |
N2b | 185 (6.1) | 131 (6.1) | 54 (6.1) | |
Surgery | 1 | |||
No | 97 (3.2) | 69 (3.2) | 28 (3.2) | |
Yes | 2,919 (96.8) | 2,064 (96.8) | 855 (96.8) | |
Scope of regional lymph node surgery | 0.631 | |||
1 to 3 | 55 (1.8) | 38 (1.8) | 17 (1.9) | |
4 or more | 2,796 (92.7) | 1,977 (92.7) | 819 (92.8) | |
None | 161 (5.3) | 114 (5.3) | 47 (5.3) | |
Sentinel node | 4 (0.1) | 4 (0.2) | 0 (0.0) | |
Radiation | 0.506 | |||
No | 2,717 (90.1) | 1,927 (90.3) | 790 (89.5) | |
Yes | 299 (9.9) | 206 (9.7) | 93 (10.5) | |
Chemotherapy | 0.246 | |||
No | 1,887 (62.6) | 1,320 (61.9) | 567 (64.2) | |
Yes | 1,129 (37.4) | 813 (38.1) | 316 (35.8) | |
CEA level† | 0.386 | |||
Negative | 1,504 (49.9) | 1,075 (50.4) | 429 (48.6) | |
Positive | 1,512 (50.1) | 1,058 (49.6) | 454 (51.4) |
†, CEA level: pretreatment recode; negative = normal/within normal limits (≤5 μg/L); positive = elevated (>5 μg/L). TNM, tumor-node-metastasis; CEA, carcinoembryonic antigen.
Feature selection and independent prognostic factors analysis
In the training set, univariate Cox regression analyses showed that all 13 variables were associated with OS in elderly patients with stage II–III (P<0.05) (Table S1). These 13 variables were considered as potential predictors. We further selected the most important features in the training set using LASSO regression algorithm. When the partial likelihood binomial deviation reaches 1se, the most appropriate tuning parameter λ for the LASSO regression was 0.0195, and ultimately 10 variables with non-zero coefficients were retained (Figure 2). The 10 variables were: age, sex, race, TNM stage, T stage, N stage, surgery, scope of regional lymph node surgery, chemotherapy and CEA level. These 10 variables were used for Cox proportional hazards analysis. Considering that T stage and N stage would interfere with TNM stage, we further excluded the variables T stage and N stage, and retained only 8 variables for Cox proportional hazards analysis. The results showed that all 8 variables were independent prognostic predictors of OS in elderly patients with stage II–III CRC (P<0.05) (Table 2). Among them, male patients possessed worse survival compared to females [hazard ratio (HR): 1.49, 95% confidence interval (CI): 1.32–1.68, P<0.001]. In stage II–III elderly CRC patients, surgery was an important treatment to improve patient survival (HR: 0.373, 95% CI: 0.234–0.595, P<0.001). There was no significant difference in OS between patients with only 1–3 lymph nodes versus without lymph node dissection (P=0.712) and only sentinel lymph node dissection (P=0.934), whereas lymph node dissection of 4 or more significantly improved patient survival (HR: 0.597, 95% CI: 0.411–0.867, P=0.007). Chemotherapy significantly improved patient prognosis (HR: 0.568, 95% CI: 0.491–0.657, P<0.001). Patients with elevated pre-treatment CEA levels had a worse prognosis (HR: 1.37, 95% CI: 1.22–1.55, P<0.001).
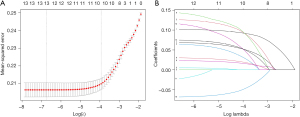
Table 2
Variables | HR | 95% CI | P value |
---|---|---|---|
Age (years) | |||
65–69 | Ref | ||
70–74 | 1.52 | 1.24–1.86 | <0.001 |
75–79 | 1.95 | 1.6–2.38 | <0.001 |
80–84 | 2.05 | 1.67–2.52 | <0.001 |
85+ | 3.8 | 3.1–4.67 | <0.001 |
Sex | |||
Female | Ref | ||
Male | 1.49 | 1.32–1.68 | <0.001 |
Race | |||
Asian or Pacific Islander | Ref | ||
Black | 1.44 | 1.27–1.64 | <0.001 |
Other | 1.21 | 0.939–1.56 | 0.14 |
TNM stage | |||
IIA | Ref | ||
IIB | 1.42 | 1.05–1.94 | 0.024 |
IIC | 2.13 | 1.54–2.94 | <0.001 |
IIIA | 1.11 | 0.813–1.52 | 0.508 |
IIIB | 1.89 | 1.63–2.2 | <0.001 |
IIIC | 3.4 | 2.8–4.13 | <0.001 |
Surgery | |||
No | Ref | ||
Yes | 0.373 | 0.234–0.595 | <0.001 |
Scope of regional lymph node surgery | |||
1 to 3 | Ref | ||
4 or more | 0.597 | 0.411–0.867 | 0.007 |
None | 0.905 | 0.533–1.54 | 0.712 |
Sentinel node | 0.941 | 0.223–3.98 | 0.934 |
Chemotherapy | |||
No | Ref | ||
Yes | 0.568 | 0.491–0.657 | <0.001 |
CEA level | |||
Negative | Ref | ||
Positive | 1.37 | 1.22–1.55 | <0.001 |
HR, hazard ratio; CI, confidence interval; TNM, tumor-node-metastasis; CEA, carcinoembryonic antigen.
Construction of the predictive nomogram and validation
Based on the results of Cox proportional hazards analysis, we constructed a survival prediction nomogram for stage II–III elderly CRC patients using the training set (Figure 3A). The online dynamic survival calculator (https://dynnomleb.shinyapps.io/JGO_ElderlyCRC_StageII-III/) were constructed based on our nomogram (Figure 3B). The nomogram could predict the 3- and 5-year survival of elderly CRC patients in stage II–III. The training set ROC curve is shown in Figure 4, the AUC value of 3-year (Figure 4A) was 76.6% (95% CI: 74.3–78.8%), and 5-year (Figure 4B) was 74.8% (95% CI: 72.6–76.9%); the AUC value of 3-year (Figure 4C) and 5-year (Figure 4D) in validation set was 72.4% (95% CI: 68.7–76.2%) and 73.1% (95% CI: 69.7–76.4%), respectively. Further internal validation of the model using k-fold cross validation (k=10) showed that the predictive model had good predictive accuracy (mean AUC =0.741).
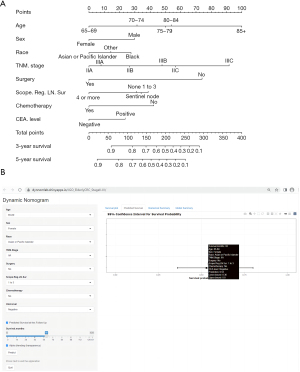
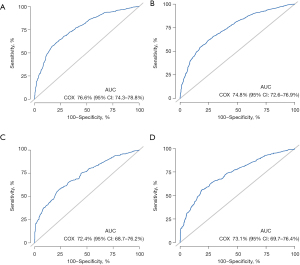
Performance of the predictive nomogram
The 3- and 5-year calibration curves in training cohort showed good performance in terms of goodness of fit (Figure 5A,5B), with a C-index of 0.707, indicating that the nomogram model performs well. The 3- and 5-year calibration curves in validation set also showed good agreement between predicted probabilities and observed probabilities (Figure 5C,5D), with a C-index of 0.682, showing acceptable discrimination, meaning that the nomogram model can accurately predict patient survival. The DCA for the training and validation sets are shown in Figure 6. In terms of 3- and 5-year OS, patients could achieve more net benefit using nomogram compared to the traditional TNM staging system. The AIC of the nomogram and TNM staging systems in the training set were 15,638.28 and 16,091.99, respectively; and in the validation set were 6,032.502 and 6,182.777, respectively. The BIC of the nomogram and TNM staging systems in the training set were 15,728.72 and 16,117.11, respectively; and in the validation set were 6,103.877 and 6,203.769, respectively. These results demonstrated that nomogram was practical and reliable.
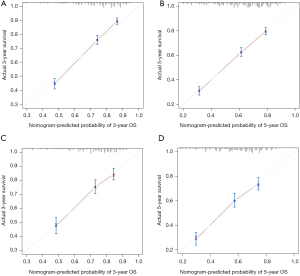
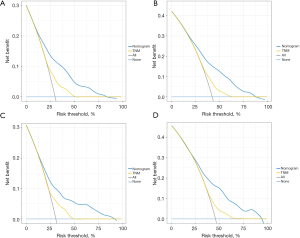
Risk stratification based on nomogram scores
Based on the constructed survival prediction nomogram, we further calculated the risk scores of all patients and selected the best cut-off value (Figure 7A). Based on the optimal cut-off value, all patients were classified into high-risk and low-risk groups. Kaplan-Meier survival analysis showed that there was a significant survival difference between high-risk and low-risk groups (Figure 7B, P<0.0001).
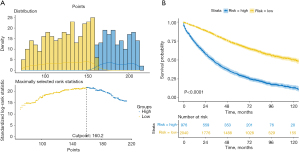
Discussion
CRC is a serious threat to human survival. With the aging of the population, more attention should be paid to the diagnosis, treatment and prognosis of the elderly CRC population.
In this study, we constructed a survival prediction nomogram for elderly CRC patients with stage II–III, which performed well and could accurately predict the survival of these patients. The AUC of the training set was 76.6% (95% CI: 74.3–78.8%), C-index: 0.707, and the AUC of the validation set was 72.4%, C-index: 0.682. The calibration curves, DCA, AIC and BIC were all performed well in the training and validation cohorts. We also found: (I) age, sex, race, TNM stage, T stage, N stage, surgery, scope of regional lymph node surgery, chemotherapy and CEA level (pretreatment record) were independent prognostic factors affecting the survival of elderly patients with stage II–III CRC, whereas radiotherapy was not; (II) surgery was a highly recommended treatment modality for elderly patients with stage II–III CRC (surgery yes vs. no: HR: 0.373, 95% CI: 0.234–0.595, P<0.001); (III) lymph node dissection in the range of 4 or more can significantly improve patient survival (HR: 0.597, 95% CI: 0.411–0.867, P=0.007, compared with 1 to 3); (IV) chemotherapy significantly improved patient survival (HR: 0.568, 95% CI: 0.491–0.657, P<0.001), but the proportion of patients receiving chemotherapy was low (37.4%); (V) elevated pre-treatment CEA level was an independent risk factor affecting elderly CRC patients with stage II–III (HR: 1.37, 95% CI: 1.22–1.55, P<0.001), but about half of the patients (49.9%) had normal pre-treatment CEA levels.
The traditional TNM staging system is widely used in the prognostic assessment of CRC patients, but it performs poorly in predicting patient survival due to its limited inclusion of features (16). To be able to accurately predict the survival of CRC patients, more and more prognostic models based on TNM staging system are being constructed. Mo et al. constructed a prognostic nomogram for CRC patients after combining clinicopathological parameters with a TNM staging system, and the results performed well (15). A CRC prognostic nomogram that introduced the Glasgow prognostic score (GPS) combined with TNM staging for the first time also showed greater advantages over traditional staging (20). However, a prognostic model that can predict stage II–III elderly CRC patients has not been constructed. Our survival prediction nomogram model, which takes into account indicators such as the patient’s pre-treatment CEA level and the type of treatment received, can accurately predict the survival of stage II–III elderly CRC patients with a prediction accuracy of 76.6%. Zhang et al. (21) constructed a well-performing prognosis nomogram of CRC patients (3-years AUC: 0.781) based on information such as modified lymph node ratio and CEA level, indicating that it is feasible to predict the survival of CRC patients based on CEA level. The nomogram of this study is the first nomogram of elderly (age >65 years) CRC patients, and the overall performance is good. Chok et al. (22) constructed a prognostic nomogram of CRC patients over the age of 80 years. Although the model performance is acceptable, its credibility is reduced due to the small sample size (n=295). Elderly cancer patients continue to face significant challenges when undergoing surgical treatment (23). Poorer nutritional status, frailty, and comorbidities remain important factors that threaten postoperative recovery in older cancer patients (24,25). Our study confirmed that surgery significantly reduced the risk of all-cause mortality (HR: 0.373, P<0.001) in stage II–III elderly CRC patients. We recommended that patients who are able to tolerate surgery should take it as far as possible. Compared with open surgery, the development of laparoscopy has brought better surgical safety and better short-term outcomes to elderly colon cancer patients (23,26). Lymph node dissection greater than 4 or more had the best prognosis for patients (HR: 0.597, P=0.007, compared with 1 to 3). However, more lymph node dissection seems to be recommended (27). Chemotherapy also significantly improved patient survival in stage II–III elderly CRC patients (HR: 0.568, P<0.001), but the proportion of chemotherapy was low (37.4%). Further promotion of chemotherapy in clinical patients appears necessary. Fluorouracil monotherapy is usually well tolerated and can provide chemotherapeutic benefits to elderly patients without a significant increase in toxicity (28-30). In our study, we also found that about half of the patients in the group of stage II–III elderly CRC patients had pre-treatment increased CEA levels, which significantly predicted a poor prognosis for the patients (HR: 1.37, 95% CI: 1.22–1.55, P<0.001). However, as a biomarker of CRC, CEA performs poorly in the detection and prognostic prediction of CRC using the CEA index alone due to its low specificity (31,32). In contrast, CEA combined with other indicators has good results (33).
In conclusion, in the future, the proportion of elderly colon cancer patients will increase yearly, and colorectal surgeons will have to face the treatment of elderly patients. It is essential to construct models that can well predict the survival of elderly CRC patients. This will help clinicians quickly identify high-risk patients and adopt more aggressive treatment strategies. We constructed a survival prediction nomogram for stage II–III elderly CRC patients, which combines factors such as pre-treatment CEA levels, and treatment modalities adopted to accurately predict patient survival. Among all factors, factors such as surgery, extent of intraoperative lymph node dissection, and chemotherapy are changeable. For some patients with high-risk scores, clinicians can actively adopt surgical treatment, chemotherapy or comprehensive treatment to reduce the risk score and ultimately extend the patient’s survival time. For patients who have been treated aggressively but still have a high-risk score, monitoring can be strengthened, postoperative management can be enhanced, and even immunotherapy can be considered to improve the patient’s prognosis (34). Although our model performs well, it has some limitations, such as not considering the pathology type of CRC patients and no external data validation. These shortcomings are also acceptable given the good performance of the model’s accuracy and the consistent performance of the training and validation sets that followed the split. It is expected that more multi-center studies can be conducted in the future to provide stronger support and raise the profile of this patient group.
Conclusions
We constructed a survival prediction nomogram for stage II–III elderly CRC patients by combining pre-treatment CEA levels, which can accurately predict patient survival. This facilitates clinicians to accurately assess patient prognosis and identify high-risk patients to adopt more aggressive and effective treatment strategies.
Acknowledgments
All authors thank SEER database for the great contributions.
Funding: This study was supported by
Footnote
Reporting Checklist: The authors have completed the TRIPOD reporting checklist. Available at https://jgo.amegroups.com/article/view/10.21037/jgo-23-863/rc
Peer Review File: Available at https://jgo.amegroups.com/article/view/10.21037/jgo-23-863/prf
Conflicts of Interest: All authors have completed the ICMJE uniform disclosure form (available at https://jgo.amegroups.com/article/view/10.21037/jgo-23-863/coif). The authors have no conflicts of interest to declare.
Ethical Statement: The authors are accountable for all aspects of the work in ensuring that questions related to the accuracy or integrity of any part of the work are appropriately investigated and resolved. The study was conducted in accordance with the Declaration of Helsinki (as revised in 2013). Ethics committee approval was not required for this study.
Open Access Statement: This is an Open Access article distributed in accordance with the Creative Commons Attribution-NonCommercial-NoDerivs 4.0 International License (CC BY-NC-ND 4.0), which permits the non-commercial replication and distribution of the article with the strict proviso that no changes or edits are made and the original work is properly cited (including links to both the formal publication through the relevant DOI and the license). See: https://creativecommons.org/licenses/by-nc-nd/4.0/.
References
- Sung H, Ferlay J, Siegel RL, et al. Global Cancer Statistics 2020: GLOBOCAN Estimates of Incidence and Mortality Worldwide for 36 Cancers in 185 Countries. CA Cancer J Clin 2021;71:209-49. [Crossref] [PubMed]
- Morgan E, Arnold M, Gini A, et al. Global burden of colorectal cancer in 2020 and 2040: incidence and mortality estimates from GLOBOCAN. Gut 2023;72:338-44. [Crossref] [PubMed]
- Xi Y, Xu P. Global colorectal cancer burden in 2020 and projections to 2040. Transl Oncol 2021;14:101174. [Crossref] [PubMed]
- Ohishi T, Kaneko MK, Yoshida Y, et al. Current Targeted Therapy for Metastatic Colorectal Cancer. Int J Mol Sci 2023;24:1702. [Crossref] [PubMed]
- Wang H, Li YJ, Lei L, et al. Estimating the economic burden of colorectal cancer in China, 2019-2030: A population-level prevalence-based analysis. Cancer Med 2023;13:e6787. [Crossref] [PubMed]
- Bhimani N, Wong GY, Molloy C, et al. Lifetime direct healthcare costs of treating colorectal cancer: a systematic review. Eur J Health Econ 2023;24:513-37. [Crossref] [PubMed]
- Dekker E, Tanis PJ, Vleugels JLA, et al. Colorectal cancer. Lancet 2019;394:1467-80. [Crossref] [PubMed]
- Gausman V, Dornblaser D, Anand S, et al. Risk Factors Associated With Early-Onset Colorectal Cancer. Clin Gastroenterol Hepatol 2020;18:2752-2759.e2. [Crossref] [PubMed]
- Angenete E. Reducing morbidity and mortality in the elderly population with colorectal cancer. Colorectal Dis 2020;22:362-3. [Crossref] [PubMed]
- Siegel RL, Wagle NS, Cercek A, et al. Colorectal cancer statistics, 2023. CA Cancer J Clin 2023;73:233-54. [Crossref] [PubMed]
- Gao Z, Wan Z, Yu P, et al. A recurrence-predictive model based on eight genes and tumor mutational burden/microsatellite instability status in Stage II/III colorectal cancer. Cancer Med 2023;13:e6720. [Crossref] [PubMed]
- Yasui K, Shida D, Ahiko Y, et al. Risk of non-colorectal cancer-related death in elderly patients with the disease: A comparison of five preoperative risk assessment indices. Cancer Med 2023;12:2290-302. [Crossref] [PubMed]
- Spaander MCW, Zauber AG, Syngal S, et al. Young-onset colorectal cancer. Nat Rev Dis Primers 2023;9:21. [Crossref] [PubMed]
- Lambregts DMJ, Bogveradze N, Blomqvist LK, et al. Current controversies in TNM for the radiological staging of rectal cancer and how to deal with them: results of a global online survey and multidisciplinary expert consensus. Eur Radiol 2022;32:4991-5003. [Crossref] [PubMed]
- Mo S, Zhou Z, Li Y, et al. Establishment and validation of a novel nomogram incorporating clinicopathological parameters into the TNM staging system to predict prognosis for stage II colorectal cancer. Cancer Cell Int 2020;20:285. [Crossref] [PubMed]
- Liu J, Huang X, Yang W, et al. Nomogram for predicting overall survival in stage II-III colorectal cancer. Cancer Med 2020;9:2363-71. [Crossref] [PubMed]
- Mahul B. Amin SBE, Frederick L. AJCC cancer staging manual. 8th ed. Greene: Springer; 2017. Available online: https://www.springer.com/us/book/9783319406176#aboutBook
- Tang G, Qi L, Sun Z, et al. Evaluation and analysis of incidence and risk factors of lower extremity venous thrombosis after urologic surgeries: A prospective two-center cohort study using LASSO-logistic regression. Int J Surg 2021;89:105948. [Crossref] [PubMed]
- Rich JT, Neely JG, Paniello RC, et al. A practical guide to understanding Kaplan-Meier curves. Otolaryngol Head Neck Surg 2010;143:331-6. [Crossref] [PubMed]
- Hong T, Cai D, Jin L, et al. Development and validation of a nomogram to predict survival after curative resection of nonmetastatic colorectal cancer. Cancer Med 2020;9:4126-36. [Crossref] [PubMed]
- Zhang ZY, Gao W, Luo QF, et al. A nomogram improves AJCC stages for colorectal cancers by introducing CEA, modified lymph node ratio and negative lymph node count. Sci Rep 2016;6:39028. [Crossref] [PubMed]
- Chok AY, Zhao Y, Chen HLR, et al. Elderly patients over 80 years undergoing colorectal cancer resection: Development and validation of a predictive nomogram for survival. World J Gastrointest Surg 2023;15:892-905. [Crossref] [PubMed]
- Navarra A, Porcellini I, Mongelli F, et al. Long-term outcomes in elderly patients after elective surgery for colorectal cancer within an ERAS protocol: a retrospective analysis. Langenbecks Arch Surg 2023;408:438. [Crossref] [PubMed]
- Martínez-Escribano C, Arteaga Moreno F, Pérez-López M, et al. Malnutrition and Increased Risk of Adverse Outcomes in Elderly Patients Undergoing Elective Colorectal Cancer Surgery: A Case-Control Study Nested in a Cohort. Nutrients 2022;14:207. [Crossref] [PubMed]
- Teo NZ, Ngu JCY. Robotic surgery in elderly patients with colorectal cancer: Review of the current literature. World J Gastrointest Surg 2023;15:1040-7. [Crossref] [PubMed]
- Fujii S, Ishibe A, Ota M, et al. Long-term results of a randomized study comparing open surgery and laparoscopic surgery in elderly colorectal cancer patients (Eld Lap study). Surg Endosc 2021;35:5686-97. [Crossref] [PubMed]
- Chen YJ, Yeh ST, Kao PS, et al. A reappraisal of lymph node dissection in colorectal cancer during primary surgical resection. World J Surg Oncol 2020;18:97. [Crossref] [PubMed]
- Biller LH, Schrag D. Diagnosis and Treatment of Metastatic Colorectal Cancer: A Review. JAMA 2021;325:669-85. [Crossref] [PubMed]
- Kim JH. Chemotherapy for colorectal cancer in the elderly. World J Gastroenterol 2015;21:5158-66. [Crossref] [PubMed]
- Audisio RA, Papamichael D. Treatment of colorectal cancer in older patients. Nat Rev Gastroenterol Hepatol 2012;9:716-25. [Crossref] [PubMed]
- Li H, Lin J, Xiao Y, et al. Colorectal Cancer Detected by Machine Learning Models Using Conventional Laboratory Test Data. Technol Cancer Res Treat 2021;20:15330338211058352. [Crossref] [PubMed]
- Kamel F, Eltarhoni K, Nisar P, et al. Colorectal Cancer Diagnosis: The Obstacles We Face in Determining a Non-Invasive Test and Current Advances in Biomarker Detection. Cancers (Basel) 2022;14:1889. [Crossref] [PubMed]
- Cai H, Chen Y, Zhang Q, et al. High preoperative CEA and systemic inflammation response index (C-SIRI) predict unfavorable survival of resectable colorectal cancer. World J Surg Oncol 2023;21:178. [Crossref] [PubMed]
- Yu I, Dakwar A, Takabe K. Immunotherapy: Recent Advances and Its Future as a Neoadjuvant, Adjuvant, and Primary Treatment in Colorectal Cancer. Cells 2023;12:258. [Crossref] [PubMed]