Disulfidptosis-related genes of prognostic signature and immune infiltration features in hepatocellular carcinoma supported by bulk and single-cell RNA sequencing data
Highlight box
Key findings
• Disulfidptosis-related genes (DRGs) have high prognostic value and play an important role in hepatocellular carcinoma (HCC) immunotherapy.
What is known, and what is new?
• The metabolism of disulfidptosis is known to be associated with treatment resistance, cancer spread, and immune system evasion in cancer cells.
• We identified that four DRGs had significant differential expression and prognostic relevance. These hub genes appear to be closely linked to HCC. We investigated variations in the tumor microenvironment, immune checkpoints, immune cell infiltration, immunotherapy, and drug sensitivity between high- and low-risk groups, and found additional evidence that DRGs can inform clinical decision-making for personalized HCC treatments.
What is the implication, and what should change now?
• Our study provides valuable insights into the identification of DRG biomarkers with potential applications for prognosis, immunotherapy, and drug sensitivity in HCC.
Introduction
Hepatocellular carcinoma (HCC) is the 6th most common cancer and the 3rd leading cause of cancer-related death worldwide (1). Despite the availability of a variety of treatment options, including surgery, targeted therapy, and immunotherapy, the 5-year overall survival (OS) of HCC patients remains poor, due to continued high rates of cancer recurrence and metastasis (2,3). The immune microenvironment is closely involved in the occurrence and progression of HCC, and different immune characteristics based on etiology have been identified (4,5). Immunotherapy has significant efficacy in treating HCC patients; however, biomarkers need to be identified to determine which patients will gain the greatest benefit. Rapid advances in bioinformatics have resulted in the development of significant techniques and platforms for screening cancer patients’ prognostic biomarkers.
Disulfidptosis is a rapid form of cell death due to disulfide stress induced by an excessive ac of cystine in glucose-deprived cells (6). The upregulation of solute carrier family 7 member 11 (SLC7A11) in kidney cancer cells enhances nicotinamide adenine dinucleotide phosphate (NADPH) depletion in the cytoplasm during glucose deprivation. This results in the accumulation of unreducible disulfides, which leads to disulfide stress and the subsequent development of disulfidptosis (6,7). Consequently, disulfide bond formation occurs between actin cytoskeleton proteins, causing the collapse of the actin filament network and ultimately resulting in disulfide ptosis. Independent of the existing cell death modes, such as apoptosis, ferroptosis, necroptosis, and cuproptosis, disulfidptosis is a novel therapeutic target in metabolic cancer and closely related to cancer. Disulfidptosis is of vital importance in the regulation of tumor development, treatment sensitivity and survival in bladder cancer (8). In addition, the metabolism of disulfides in cancer cells has been linked to a number of different biological phenomena, including drug resistance, the spread of cancer, and the ability of cancer to evade the immune system (9). Programmed cell death is closely related to immunity (10). Nevertheless, the function of disulfidptosis in tumor immune response, immune infiltration, and immunotherapy is not yet known. Activating disulfidptosis pathways could overcome patients’ resistance to current chemotherapeutics and pave the way for new cancer treatments. The interrelationship between disulfidptosis-associated genes and the clinical outcomes of HCC patients must be thoroughly investigated. Thus, we conducted this study to examine the effects of disulfidptosis on HCC prognosis and immunization.
We analyzed the functions of genes associated with disulfidptosis using RNA-sequencing (RNA-seq) and single cell RNA sequencing (scRNA-seq) data from The Cancer Genome Atlas–Liver Hepatocellular Carcinoma (TCGA-LIHC), GSE76427, and GSE98638 data sets. Four gene regulators associated with disulfidptosis were selected to evaluate HCC. Based on these findings, a risk model and nomogram were developed that could provide significant clinical benefits for HCC patients. Additionally, two risk categories were identified, and significant associations were observed between these categories, immune cell infiltration, and prospective medication selection. In conclusion, a deeper understanding of disulfidptosis processes will facilitate the evolution of new molecular therapies and inform the future immunotherapy strategies for HCC. We present this article in accordance with the TRIPOD reporting checklist (available at https://jgo.amegroups.com/article/view/10.21037/jgo-23-949/rc).
Methods
Data acquisition
The RNA-seq and clinical and mutation data of HCC patients were extracted from TCGA database (https://portal.gdc.cancer.gov/). We used a data set comprising 374 HCC and 50 normal liver samples for the differential analysis, excluding samples without clinical data or with an OS less than 30 days. As an external validation data set, 115 HCC samples were obtained from GSE76427 from the Gene Expression Omnibus (GEO) database (https://www.ncbi.nlm.nih.gov/geo). To investigate the expression of disulfidptosis-related genes (DRGs) in the tumor microenvironment (TME) at the single-cell level, we employed the Tumor Immune Single Cell Center (TISCH) (11). Previous studies have identified 24 DRGs (6,12,13). The flow chart for the data processing is shown in Figure 1. This study was conducted in accordance with the Declaration of Helsinki (as revised in 2013).
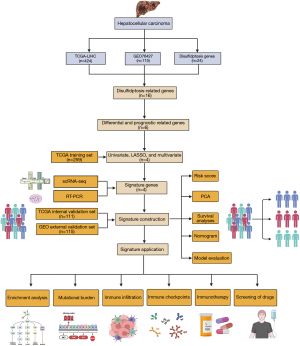
Construction and analysis of DRGs signature
TCGA database was used to filter the differentially expressed genes (DEGs) associated with disulfidptosis between the tumor and normal samples using the “DESeq2” package (Michael, 2014) utility [threshold: false discovery rate (FDR) >0.05, |log2(fold change)| >1]. The tumor samples were randomly divided into training and testing cohorts at a 7:3 ratio using the “caret” package (Kuhn, 2008). A univariate analysis, least absolute shrinkage and selection operator (LASSO) analysis, and multivariate logistic regression analysis were performed using the “glmnet” (Friedman, 2010) and “survival” packages (Therneau, 2022) in R. A P value <0.5 was set as the significance threshold for genes in the univariate logistic regression. Using the following formula, we calculated a risk score for each LIHC sample based on these gene expression levels: Risk score = β1 × gene1 + β2 × gene2 + β3×gene3 + ... + βn × geneN. Based on the median risk score, the patients in the training set were divided into high- and low-risk groups. Kaplan-Meier survival curves were generated using the “survival” package (Therneau, 2022) to calculate the OS of each cohort. Using time-dependent receiver operating characteristic (ROC) curve profiles at 1, 3, and 5 years, the model’s accuracy was evaluated. Comparisons were made between the two risk groups in relation to the distribution of risk score, patient survival, and gene expression. Further, the model was validated using the testing cohort, total cohort, and an external validation set. A nomogram was established that incorporated the risk score, age, gender, and pathological stage as prognostic factors. Calibration and ROC curves were generated to appraise the accuracy of the nomogram. A stratified analysis was conducted to assess the prognostic significance of the risk score. The validation set was treated the same as the test set in terms of its settings, inclusion criteria, outcomes, and predictors.
Analysis of biological characteristics between the distinct risk groups
Gene Ontology (GO) is commonly used to interpret gene lists in enrichment analyses (14). The Kyoto Encyclopedia of Genes and Genomes (KEGG) is a comprehensive pathway-oriented knowledge base that includes 15 databases covering 3,982 organisms (15). We used the “clusterProfiler” package (T, 2021) in R to analyze GO and KEGG to gain a better understanding of the potential mechanisms of the DEGs in HCC. A filtering criterion of an FDR <0.05 was established. A gene set enrichment analysis (GSEA) was conducted to identify the significantly different biological processes between the clusters. A GSEA is commonly used to evaluate the statistical significance of differences between two biological processes. The GSEA was performed using “c2.cp.kegg.v7.4.symbols.gmt” and “c5.go.v7.2.symbols.gmt” obtained from the Molecular Signature Database (MSigDB).
Somatic mutation analysis
R’s “maftools” package (Mayakonda, 2018) was used to visualize the mutation data from LIHC. Fisher’s exact test was employed to evaluate the co-mutation of genes. The tumor mutation burden (TMB) was estimated by considering non-synonymous and code-shifting indels, with a detection limit below 5%. The TMB represents the count of somatic, coding, base substitution, and insert-deletion variants per megabase of the genome.
Immune landscapes related to the DRGs
Using the “ESTIMATE” package (Yoshihara, 2016), stromal scores and immune scores were calculated for each TCGA-LIHC sample to estimate tumor purity. Based on the absolute abundance of immune cells and stromal cells, a “CIBERSORT” package (Aaron, 2015) analysis was conducted to estimate the percentages of 22 human immune cell categories. A Spearman analysis was performed to determine the correlation coefficient between the infiltrating immune cells and the risk score, and the results were visualized on a scatter diagram. The expression of genes involved in the immune checkpoints were correlated with the response to immune checkpoint inhibitors (ICIs), and immune checkpoint gene expression was compared between the two risk groups. Additionally, the Spearman correlation analysis identified the DRGs that were most strongly associated with the immune checkpoints. The IMvigor210 cohort was chosen to validate the immune checkpoint blockade treatment.
Drug sensitivity analysis
The “oncoPredict” package (Maeser, 2021) was used to identify targeted medications and assess whether they were significantly correlated with the risk score to investigate potential therapeutic strategies. Semi-inhibitory concentrations [e.g., a half-maximal inhibitory concentration (IC50)] were determined and compared using the Wilcoxon signed-rank test.
Real-time quantitative PCR (RT-qPCR)
The total RNA from the experimental and control groups was extracted using the RNA rapid purification reagent (ES Science) following the manufacturer’s instructions. RNA concentration and purity were detected using the Nano-Drop One instrument (Thermo Fisher Scientific, Waltham, MA, USA). The reverse transcription of complementary DNA was carried out using s1000 (Bio-Rad Laboratories, Hercules, CA, USA). qRT-PCR was conducted with SYBR Green (Yeasen Biotech Co, Shanghai, China). The sequences of the RT-qPCR primers are set out in Table 1. Gene expression was determined using the 2−ΔΔCt method. β-actin messenger RNA served as the internal normal reference.
Table 1
Gene | Forward primer (5'-3') | Reverse primer (5'-3') |
---|---|---|
β-actin | TCCCTGGAGAAGAGCTACGA | AGCACTGTGTTGGCGTACAG |
RPN1 | GGCCAAGATTTCAGTCATTGTGG | CTTCGTTGGATAGGGAGAGTAGA |
SLC2A1 | GGCCAAGAGTGTGCTAAAGAA | ACAGCGTTGATGCCAGACAG |
SLC2A4 | TGGGCGGCATGATTTCCTC | GCCAGGACATTGTTGACCAG |
SLC7A11 | TCTCCAAAGGAGGTTACCTGC | AGACTCCCCTCAGTAAAGTGAC |
Statistical analysis
The statistical analyses were conducted using R (version 4.2.2, ucrt). Each in vitro experiment was conducted a minimum of three times. GraphPad Prism (version 8.0, San Diego, CA, USA) was used to conduct the statistical analyses. Student’s t-tests were used for the continuous variables with a normal distribution and Wilcoxon rank-sum tests were used for those with a non-normal distribution. The Chi-square or Fisher’s exact test was used for the categorical variables as applicable. A Pearson analysis was carried out to assess the correlations. Two-tailed P values were calculated, and statistical significance was defined as a P value <0.05.
Results
Identification of differentially expressed DRG signature
Both the training cohort and the internal validation cohort comprised tumor samples from the TCGA-LIHC data set. For external validation, the GSE76427 data set from the GEO database, which comprised 115 tumor samples, was used. Previous research identified 24 DRGs (6,12,13). A volcano plot shows the expression changes of all the DRGs in the HCC samples (Figure 2A). By cross-referencing the three data sets, we identified 16 differentially expressed DRGs that were highly expressed in the tumor tissues (Figure 2B). The prognostic analysis showed that five genes were associated with a favorable prognosis, while one gene was associated with a poor prognosis (Figure 2C). The results of a network interaction analysis (Figure 2D) shows the risk factors in purple and favorable prognostic factors in green. To better understand the role of the DRGs in HCC, the TCGA-LIHC data set was divided into a training and validation set at a ratio of 7:3. Six prognostic genes were identified through the univariate Cox regression analysis. A LASSO regression analysis was then conducted to confirm that the genes were significantly correlated with OS in HCC (Figure 2E). The selected genes underwent model cross-validation (Figure 2F). The prognostic DRGs were then identified using a multivariate Cox regression analysis. Among the DRGs in the training set, RPN1, SLC2A1, SLC2A4, and SLC7A11 were found to be prognostic genes associated with OS. These genes were used to identify predictive characteristics for patients in the LIHC training set.
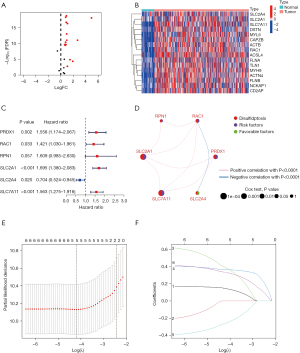
Establishment of a prognostic model
The HCC patients in the training, testing, entire, and external validation set were stratified into high- and low-risk groups according to the median value of the predictive risk score. The principal component analysis results showed that the four DRGs could effectively differentiate between the high- and low-risk categories across multiple data sets (Figure 3A). Figure 3B illustrates the distribution of the prognostic signature, while Figure 3C shows the survival outcomes of patients in different categories. Figure 3D illustrates the expression profiles of the four DRGs. Figure 3E shows that compared with the high-risk group, the survival of HCC patients in the low-risk group was significantly longer (P<0.05). To determine the predictive ability of the model, we depicted the ROC curve to validate the Kaplan-Meier results, and a higher area under the curve (AUC) indicated better performance. These genes exhibited excellent performance in our predictive model (Figure 3F). These findings indicate that prognostic features can be used to differentiate between high- and low-risk groups.
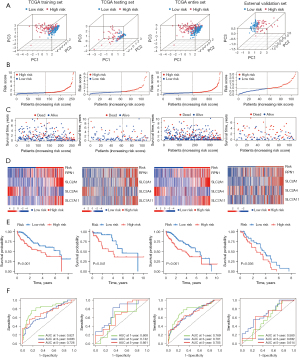
Establishment and evaluation of a clinical nomogram
The multivariate Cox regression analysis showed that risk score and tumor stage were independent risk factors in HCC (Figure 4A). Additionally, we constructed a nomogram to calculate the individual survival probabilities of patients at 1, 3, and 5 years (Figure 4B). A calibration plot of the nomogram was used to assess the accuracy and sensitivity of the prediction results, and a good correlation was found between the actual and predicted survival rates at 1, 3, and 5 years (Figure 4C). The ROC curve indicated that the nomogram achieved a 1-year AUC of 0.794, surpassing other clinical parameters (risk, AUC =0.782; age, AUC =0.634; gender, AUC =0.500; stage, AUC =0.500; Figure 4D-4F). Based on the results, the nomogram effectively predicted the OS of HCC patients.
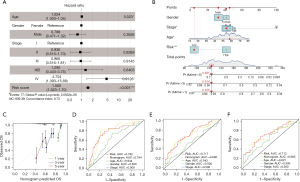
Functional enrichment analysis
Given the favorable prognostic performance of the DRGs signature in the HCC patients, we then sought to investigate the underlying mechanism. Initially, a differential expression analysis between the two risk groups was performed (|log2 fold change| ≥0.5, adjusted P value <0.05). The DEGs were subsequently analyzed using GO and KEGG methods. The analysis of the biological processes revealed an overrepresentation of DEGs in the cellular cycle, DNA replication, and metabolic pathways (Figure 5A,5B). Additionally, a GSEA was used to investigate the substantially enriched functional terms between patients at high risk and those at low risk. DNA repair, cell division, and cancer pathways were significantly enriched in the high-risk group (Figure 5C,5D). The above findings suggest that metabolic activity and drug metabolism may be the underlying mechanisms behind the predictive ability of our DRGs-based signature for HCC patient prognosis.
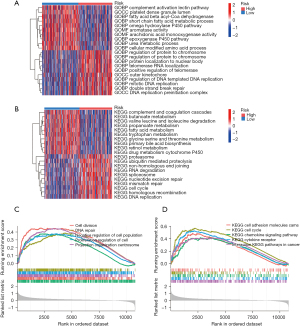
DRGs association with TMB
The high-risk group had significantly higher frequencies of somatic mutations than the low-risk group, particularly in TP53 (41% vs. 17%), CSMD3 (13% vs. 4%), and ZNF831 (6% vs. 1%) (Figure 6A,6B). The high-risk patients had higher TMB levels than the low-risk patients (Figure 6C). Following the integration of the TMB scores, the HCC patients from TCGA were categorized into four groups. The survival analysis demonstrated that patients with a low TMB and a low risk had a significant survival advantage, followed by patients with a high TMB and a low risk, while patients with a high TMB and a high risk had the worst survival prospects. The group with a low TMB and a low risk had a significant survival disadvantage (Figure 6D). The disparity in the TMB between the two groups could provide insights for future treatment strategies.
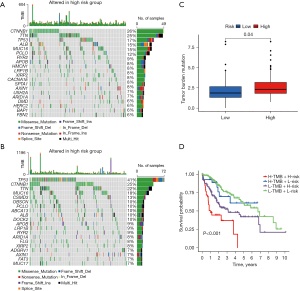
Assessment of TME and forecasting immunotherapy
Tumor growth, response to therapy, and clinical outcomes are all significantly affected by stromal cells and immune cells inside the TME (16,17). The violin plot depicts the stromal score and immune activity of each sample. We observed a relatively higher abundance of stromal cells in the high-risk group of DRGs (Figure 7A). Additionally, the immune scores of the high-risk group of DRGs were higher than those of the low-risk group (Figure 7A). To conduct a comprehensive analysis of the immune microenvironment, we calculated the infiltration levels of 22 immune cell types using “CIBERSORT” package (Aaron, 2015). Figure 7B illustrates the immune landscape of the TCGA-LIHC samples. Follicular helper T cells, regulatory T (Treg) cells, M0 macrophages, M2 macrophages, and quiescent mast cells exhibited significant increases in the high-risk DRG group, as evidenced by the comparison of immune cell profiles. Conversely, memory cluster of differentiation CD4 T cells, and mast cells were more abundant in the low-risk group (Figure 7B). We also investigated the relationship between immunocytography and DRGs scores. We found that the DRGs score was positively correlated with activated M0 macrophages, memory CD4 T cells, and other immune cells (Figure 7C). In TCGA cohort, the expression levels of multiple immune checkpoints were significantly elevated in the high-risk group (Figure 8A), implying that high-risk patients might benefit more from ICI therapy. The heatmap showed that the DRGs were closely related to immune checkpoints (Figure 8B). Lastly, IMvigor210 was used to validate the prognostic and immunotherapeutic efficacy of the risk score (Figure 8C). The effectiveness of immunotherapy was enhanced in individuals with low-risk scores.
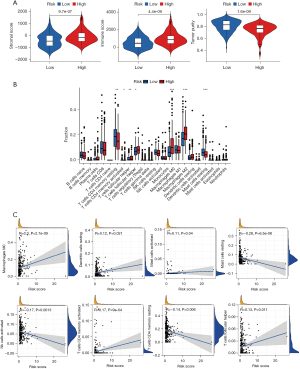
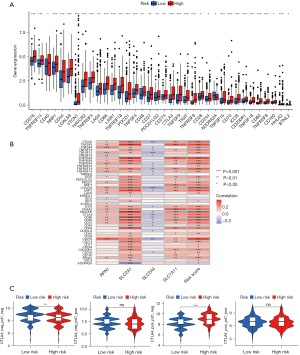
Potential drug screening
The “Oncopredict” package (Maeser, 2021) was used to assess the IC50 values of commonly used pharmaceuticals. The high-risk group exhibited decreased IC50 values for sorafenib, zoledronate, vorinostat, nilotinib, carmustine, ruxolitinib, and cyclophosphamide (Figure 9A-9C). We further investigated the association between DRGs and IC50 using scatterplots (Figure 9B-9D). A lower IC50 value indicated greater drug sensitivity and therapeutic efficacy.
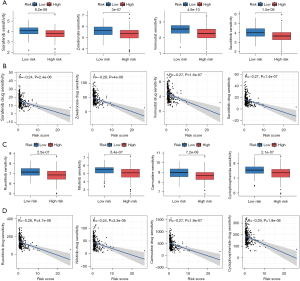
ScRNA-Seq analysis
TISCH was employed to evaluate the expression levels of DRGs in the tumor immune cells and to investigate the role of DRGs in these cells. Figure 10A displays the cells divided into 16 clusters, while Figure 10B provides annotations for the immune cell types. Both the pie chart and bar chart showed that CD4 T conventional cells had the highest proportion, followed by CD8 T cells (Figure 10C,10D). CD4 T conventional cells , CD8 T cells, CD8 T exhaustion cell, T proliferation cell , and Treg cells exhibited high expression levels of RPN1 and SLC2A1. Moreover, SLC2A1 was specifically expressed in Treg cells, while CD8 T exhaustion cell, T proliferation cell, and Treg cells showed characteristic expression of SLC7A11 (Figure 10E,10F).
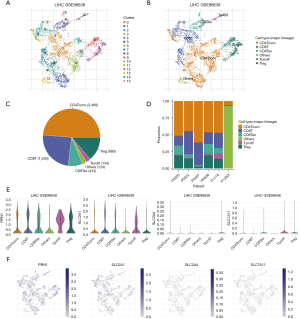
Validation of the four DRGs
To experimentally validate our findings, we performed a RT-qPCR analysis and observed the differential expression of four DRGs between the normal liver cell line (MIHA) and HCC cell lines (Hep3B, Huh7, MHCC97H, and SNU387). The expression levels of RPN1, SLC2A1, SLC2A4, and SLC7A11 were significantly upregulated in the HCC cells compared to MIHA cells (Figure 11A-11D).

Discussion
Despite encouraging progress being achieved in the detection, treatment, and prevention of HCC, it remains a prevalent and malignant disease with a high mortality rate and a poor prognosis, resulting in over 500,000 deaths annually (18,19). HCC represents 90% of all kind of liver cancers, and its 5-year survival rate is only 18% (1,20). Typically, patients diagnosed with early-stage HCC receive standard treatments, including surgical resection, radiofrequency ablation, and transarterial chemoembolization (21). Chemotherapy resistance is widely acknowledged as a leading cause of mortality in cancer patients (22,23). Recent diagnostic and therapeutic improvements have not improved the prognosis of patients with HCC. Thus, innovative medicines and reliable biomarkers need to be quickly identified to address this issue.
Due to the drug-resistance of tumor cells, researchers have focused on programmed cell death mechanisms. Disulfidptosis, a novel form of cell death that occurs when disulfide are increased in cancer cells, and thus may offer a potential therapeutic strategy for cancer (24). Nevertheless, the use of immunotherapy as a primary treatment approach has challenged the position of sorafenib (25). We investigated the influence of disulfidptosis on the immune response in HCC. We identified the prognostic features of DRGs using TCGA-LIHC data set. Subsequently, we corroborated discrepancies in tumor-infiltrating immune cells and immune checkpoints using a newly developed prognostic signature.
In the study, we confirmed the significance of DRGs in HCC through a bioinformatics analysis of RNA-seq and scRNA-seq. For the investigation of prognostic indicators related to disulfidptosis, we extracted six DRGs from TCGA data set. TCGA data set validated the predictive value of six DRGs, and four of these genes were used to build prognostic signatures to predict the OS of HCC patients. Among the six genes, four were identified (i.e., RPN1, SLC2A1, SLC2A4, and SLC7A11).
A study has shown that the knockdown of RPN1 in vitro inhibits breast cancer cells proliferation, as well as induces cell apoptosis via endoplasmic reticulum stress (26). SLC2A1 promotes glycolysis, proliferation and migration of colorectal cancer (27). The SLC2A4 level is significantly correlated with to the prognosis of breast cancer patients (28). SOCS2 acts as a bridge, facilitating the transfer of ubiquitin to SLC7A11, thereby promoting K48-linked polyubiquitination and the subsequent degradation of SLC7A11, and this process triggers the onset of ferroptosis and enhances radiosensitivity in HCC (29). Nevertheless, there is a lack of published studies investigating the correlation between these four DRGs and HCC. Our research may provide valuable insights for future experimental investigations.
The four selected genes were used to develop a prognostic signature. Patients were allocated to high- and low-risk groups based on the median score. The high-risk group exhibited worse clinical outcomes than the low-risk group. The DRGs prognostic signature was identified as an independent risk factor for HCC prognosis in the multivariate Cox regression analysis. The ROC analysis indicated that the signature surpassed conventional clinical characteristics in predicting HCC patient survival. Further, a nomogram was developed to examine the optimal consistency of the 1-, 3-, and 5-year prediction rates. The DRGs signature is reliable, accurate, and capable of identifying novel biomarkers for future research.
We identified DEGs between the high- and low-risk groups to investigate the aberrant changes in the downstream pathway. The GO and KEGG enrichment analysis results showed that the identified DEGs were mainly associated with cell cycle, DNA replication, and metabolic processes. Further, the GSEA demonstrated the significant enrichment of the DEGs in DNA repair, cell division, and cancer-related pathways. All of the aforementioned enriched pathways were strongly associated with the development of malignancies.
There is increasing evidence that immune cell infiltration is crucial to the initiation and progression of cancer, leading to adverse effects on clinical prognosis and treatment efficacy (30,31). This study found a strong correlation between disulfidptosis and the extent of immune cell infiltration in HCC, which adds to the importance of our findings. The expression of DRGs was found to be significantly correlated with the abundance of follicular helper T cells, Treg cells, M0 macrophages, M2 macrophages, and resting mast cells.
T helper (Th) cells play a crucial role in immune regulation, and the balance between Th1 and Th2 subsets is tightly controlled under physiological conditions (32). Imbalances in the Th1/Th2 ratio occur when the production of Th2 cytokines is elevated, leading to a shift towards a Th2-dominant state (33). Numerous malignancies, such as HCC, lung cancer, and gastric cancer, exhibit a Th1/Th2 imbalance marked by an abundance of Th2 cells, possibly contributing to tumor immune escape mechanisms (34). Through immune monitoring and immune evasion mechanisms, the human immune system plays a vital role in cancer progression (35,36).
The TME is primarily characterized by the presence of M2-polarized tumor-associated macrophages that secrete immunosuppressive cytokines and facilitate angiogenesis, tumor growth, and metastasis (37,38). Consistent with previous findings, our study discovered a positive correlation between the expression of DRGs and the infiltration of M0 macrophages and Th cells in HCC. Lastly, a single-cell data analysis was carried out to evaluate the expression of DRGs in immune cells. The correlation between disulfidptosis and immune cell infiltration, along with expression patterns and prognostic significance, indicated a potential mechanism involving collaborative interactions between Th cells and macrophages in the development of HCC.
Our study identified distinct immunological characteristics in two risk groups, suggesting the potential for individualized immunotherapy and targeted therapy based on these characteristics. Further, we assessed drug sensitivity based on disulfide-linked genes in HCC patients. Accurate predictions could streamline drug discoveries and development processes (39), while providing a comprehensive understanding of drug mechanisms of action (40). We also conducted in vitro studies to confirm the levels of DRGs expression in HCC cells.
Despite the fact that our study discovered the possible significance and the mechanism of disulfidptosis in HCC progression, it is essential to acknowledge its limitations. First, the functional evaluation of disulfidptosis was performed using a vitro model, which has not been validated in vivo; thus, further research is required. Second, the expression of disulfidptosis should be validated in cells to mitigate potential errors associated with the use of publicly available data sets. Third, beyond disulfidptosis, other cell death mechanisms, including apoptosis, ferroptosis, necroptosis, and cuproptosis, are intricately linked to liver cancer and warrant further investigation. Fourth, the model we have developed is based on some DRGs genes, but the potential of other DRGs in liver cancer research remains to be investigated. This study demonstrated that disulfidptosis has a regulatory effect on the cell cycle and immunological invasion; however, the underlying molecular mechanisms have yet to be explored.
Conclusions
In this study, we examined the expression of DRGs in HCC. A disulfidptosis model was developed to enhance prognostic risk stratification. Further, we conducted a comprehensive analysis of the clinical characteristics, risk score, immune cell infiltration, immune microenvironment, drug sensitivity, and experimental validation of four DRGs to assess their effect on HCC. In conclusion, we investigated the prognostic value of DRGs and their implications for immunotherapy in patients with HCC.
Acknowledgments
We are grateful for the information provided by public data sets such as TCGA and GEO.
Funding: This study was supported by funding from
Footnote
Reporting Checklist: The authors have completed the TRIPOD reporting checklist. Available at https://jgo.amegroups.com/article/view/10.21037/jgo-23-949/rc
Peer Review File: Available at https://jgo.amegroups.com/article/view/10.21037/jgo-23-949/prf
Conflicts of Interest: All authors have completed the ICMJE uniform disclosure form (available at https://jgo.amegroups.com/article/view/10.21037/jgo-23-949/coif). The authors have no conflicts of interest to declare.
Ethical Statement: The authors are accountable for all aspects of the work in ensuring that questions related to the accuracy or integrity of any part of the work are appropriately investigated and resolved. This study was conducted in accordance with the Declaration of Helsinki (as revised in 2013).
Open Access Statement: This is an Open Access article distributed in accordance with the Creative Commons Attribution-NonCommercial-NoDerivs 4.0 International License (CC BY-NC-ND 4.0), which permits the non-commercial replication and distribution of the article with the strict proviso that no changes or edits are made and the original work is properly cited (including links to both the formal publication through the relevant DOI and the license). See: https://creativecommons.org/licenses/by-nc-nd/4.0/.
References
- Sung H, Ferlay J, Siegel RL, et al. Global Cancer Statistics 2020: GLOBOCAN Estimates of Incidence and Mortality Worldwide for 36 Cancers in 185 Countries. CA Cancer J Clin 2021;71:209-49. [Crossref] [PubMed]
- Kanno H, Goto Y, Sasaki S, et al. Geriatric nutritional risk index predicts prognosis in hepatocellular carcinoma after hepatectomy: a propensity score matching analysis. Sci Rep 2021;11:9038. [Crossref] [PubMed]
- Llovet JM, De Baere T, Kulik L, et al. Locoregional therapies in the era of molecular and immune treatments for hepatocellular carcinoma. Nat Rev Gastroenterol Hepatol 2021;18:293-313. [Crossref] [PubMed]
- Murai H, Kodama T, Maesaka K, et al. Multiomics identifies the link between intratumor steatosis and the exhausted tumor immune microenvironment in hepatocellular carcinoma. Hepatology 2023;77:77-91. [Crossref] [PubMed]
- Donne R, Lujambio A. The liver cancer immune microenvironment: Therapeutic implications for hepatocellular carcinoma. Hepatology 2023;77:1773-96. [Crossref] [PubMed]
- Liu X, Nie L, Zhang Y, et al. Actin cytoskeleton vulnerability to disulfide stress mediates disulfidptosis. Nat Cell Biol 2023;25:404-14. [Crossref] [PubMed]
- Zheng P, Zhou C, Ding Y, et al. Disulfidptosis: a new target for metabolic cancer therapy. J Exp Clin Cancer Res 2023;42:103. [Crossref] [PubMed]
- Chen H, Yang W, Li Y, et al. Leveraging a disulfidptosis-based signature to improve the survival and drug sensitivity of bladder cancer patients. Front Immunol 2023;14:1198878. [Crossref] [PubMed]
- Wang Y, Jiang Y, Wei D, et al. Nanoparticle-mediated convection-enhanced delivery of a DNA intercalator to gliomas circumvents temozolomide resistance. Nat Biomed Eng 2021;5:1048-58. [Crossref] [PubMed]
- Liu J, Hong M, Li Y, et al. Programmed Cell Death Tunes Tumor Immunity. Front Immunol 2022;13:847345. [Crossref] [PubMed]
- Sun D, Wang J, Han Y, et al. TISCH: a comprehensive web resource enabling interactive single-cell transcriptome visualization of tumor microenvironment. Nucleic Acids Res 2021;49:D1420-30. [Crossref] [PubMed]
- Feng Z, Zhao Q, Ding Y, et al. Identification a unique disulfidptosis classification regarding prognosis and immune landscapes in thyroid carcinoma and providing therapeutic strategies. J Cancer Res Clin Oncol 2023;149:11157-70. [Crossref] [PubMed]
- Xue W, Qiu K, Dong B, et al. Disulfidptosis-associated long non-coding RNA signature predicts the prognosis, tumor microenvironment, and immunotherapy and chemotherapy options in colon adenocarcinoma. Cancer Cell Int 2023;23:218. [Crossref] [PubMed]
- Peng C, Zhang Y, Lang X, et al. Role of mitochondrial metabolic disorder and immune infiltration in diabetic cardiomyopathy: new insights from bioinformatics analysis. J Transl Med 2023;21:66. [Crossref] [PubMed]
- Kanehisa M, Furumichi M, Sato Y, et al. KEGG for taxonomy-based analysis of pathways and genomes. Nucleic Acids Res 2023;51:D587-92. [Crossref] [PubMed]
- Bejarano L, Jordāo MJC, Joyce JA. Therapeutic Targeting of the Tumor Microenvironment. Cancer Discov 2021;11:933-59. [Crossref] [PubMed]
- Pan K, Farrukh H, Chittepu VCSR, et al. CAR race to cancer immunotherapy: from CAR T, CAR NK to CAR macrophage therapy. J Exp Clin Cancer Res 2022;41:119. [Crossref] [PubMed]
- Antkowiak M, Gabr A, Das A, et al. Prognostic Role of Albumin, Bilirubin, and ALBI Scores: Analysis of 1000 Patients with Hepatocellular Carcinoma Undergoing Radioembolization. Cancers (Basel) 2019;11:879. [Crossref] [PubMed]
- Cai C, Zhang Y, Hu X, et al. Corrigendum: CDT1 is a Novel Prognostic and Predictive Biomarkers for Hepatocellular Carcinoma. Front Oncol 2021;11:801970. [Crossref] [PubMed]
- Cronin KA, Scott S, Firth AU, et al. Annual report to the nation on the status of cancer, part 1: National cancer statistics. Cancer 2022;128:4251-84. [Crossref] [PubMed]
- Zhang B, Zhao J, Liu B, et al. Development and Validation of a Novel Ferroptosis-Related Gene Signature for Prognosis and Immunotherapy in Hepatocellular Carcinoma. Front Mol Biosci 2022;9:940575. [Crossref] [PubMed]
- McMonnies CW, Ho A. Patient history in screening for dry eye conditions. J Am Optom Assoc 1987;58:296-301. [PubMed]
- Yang C, Zhang H, Zhang L, et al. Evolving therapeutic landscape of advanced hepatocellular carcinoma. Nat Rev Gastroenterol Hepatol 2023;20:203-22. [Crossref] [PubMed]
- Zhao S, Wang L, Ding W, et al. Crosstalk of disulfidptosis-related subtypes, establishment of a prognostic signature and immune infiltration characteristics in bladder cancer based on a machine learning survival framework. Front Endocrinol (Lausanne) 2023;14:1180404. [Crossref] [PubMed]
- Llovet JM, Castet F, Heikenwalder M, et al. Immunotherapies for hepatocellular carcinoma. Nat Rev Clin Oncol 2022;19:151-72. [Crossref] [PubMed]
- Ding J, Xu J, Deng Q, et al. Knockdown of Oligosaccharyltransferase Subunit Ribophorin 1 Induces Endoplasmic-Reticulum-Stress-Dependent Cell Apoptosis in Breast Cancer. Front Oncol 2021;11:722624. [Crossref] [PubMed]
- Liu XS, Yang JW, Zeng J, et al. SLC2A1 is a Diagnostic Biomarker Involved in Immune Infiltration of Colorectal Cancer and Associated With m6A Modification and ceRNA. Front Cell Dev Biol 2022;10:853596. [Crossref] [PubMed]
- Shi Z, Liu J, Wang F, et al. Integrated analysis of Solute carrier family-2 members reveals SLC2A4 as an independent favorable prognostic biomarker for breast cancer. Channels (Austin) 2021;15:555-68. [Crossref] [PubMed]
- Chen Q, Zheng W, Guan J, et al. SOCS2-enhanced ubiquitination of SLC7A11 promotes ferroptosis and radiosensitization in hepatocellular carcinoma. Cell Death Differ 2023;30:137-51. [Crossref] [PubMed]
- Yang Z, Yan G, Zheng L, et al. YKT6, as a potential predictor of prognosis and immunotherapy response for oral squamous cell carcinoma, is related to cell invasion, metastasis, and CD8+ T cell infiltration. Oncoimmunology 2021;10:1938890. [Crossref] [PubMed]
- Fang P, Zhou J, Liang Z, et al. Immunotherapy resistance in esophageal cancer: Possible mechanisms and clinical implications. Front Immunol 2022;13:975986. [Crossref] [PubMed]
- Xiao Y, Huang Y, Jiang J, et al. Identification of the prognostic value of Th1/Th2 ratio and a novel prognostic signature in basal-like breast cancer. Hereditas 2023;160:2. [Crossref] [PubMed]
- Zhao X, Liu J, Ge S, et al. Saikosaponin A Inhibits Breast Cancer by Regulating Th1/Th2 Balance. Front Pharmacol 2019;10:624. [Crossref] [PubMed]
- Zheng Z, Li YN, Jia S, et al. Lung mesenchymal stromal cells influenced by Th2 cytokines mobilize neutrophils and facilitate metastasis by producing complement C3. Nat Commun 2021;12:6202. [Crossref] [PubMed]
- Shen L, Zhou Y, He H, et al. Crosstalk between Macrophages, T Cells, and Iron Metabolism in Tumor Microenvironment. Oxid Med Cell Longev 2021;2021:8865791. [Crossref] [PubMed]
- Zhou J, Tang Z, Gao S, et al. Tumor-Associated Macrophages: Recent Insights and Therapies. Front Oncol 2020;10:188. [Crossref] [PubMed]
- Fang W, Zhou T, Shi H, et al. Progranulin induces immune escape in breast cancer via up-regulating PD-L1 expression on tumor-associated macrophages (TAMs) and promoting CD8(+) T cell exclusion. J Exp Clin Cancer Res 2021;40:4. [Crossref] [PubMed]
- Zhao L, Ning Q, Zheng G, et al. exRNAdisease: An extracellular RNA transcriptome atlas in human diseases. Gene 2022;836:146662. [Crossref] [PubMed]
- Decherchi S, Cavalli A. Thermodynamics and Kinetics of Drug-Target Binding by Molecular Simulation. Chem Rev 2020;120:12788-833. [Crossref] [PubMed]
- Chen H, Cheng F, Li J. iDrug: Integration of drug repositioning and drug-target prediction via cross-network embedding. PLoS Comput Biol 2020;16:e1008040. [Crossref] [PubMed]